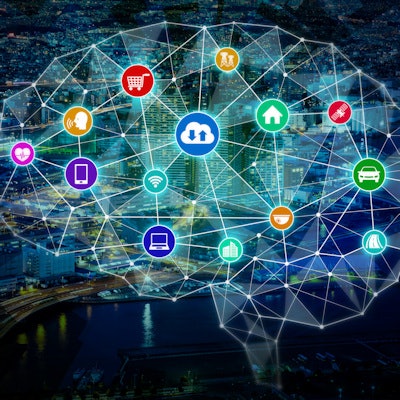
Artificial intelligence (AI) is not yet ready for routine adoption, and radiologists must develop new skills to work effectively in a machine-learning environment, a U.S. expert told delegates at the recent congress of the European Society of Medical Imaging Informatics (EuSoMII) in Rotterdam, the Netherlands.
Developers of AI face difficulties building machine-learning algorithms that generalize enough to work well in different settings, according to Dr. Raym Geis, senior scientist at the American College of Radiology (ACR) Data Science Institute and associate professor of radiology at National Jewish Health hospital in Denver.
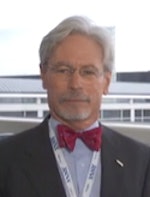
He described how the state of machine-learning research and technology, and the discussion about using machine learning for radiology computer vision, have diverged: While the promise of machine learning is great, its actual abilities today may not match the hype commonly seen in the press, he stressed.
"To quote Aleksander Madry, a computer science professor at Massachusetts Institute of Technology, 'We should be careful about machine learning because the truth -- as unpopular as it might be -- is that machine learning is not yet ready for the real-world deployment it is currently undergoing,' " Geis told delegates.
Although not yet ready for routine application, this is not because machine learning doesn't work, he noted.
"In actual fact, machine learning works surprisingly well, better than we might have first expected, but it works in settings with very consistent, standardized data. Another way to describe this is it's not that machine learning doesn't work; it's that machine learning for radiology exam analysis doesn't generalize," he noted in his presentation, AI, what it is -- and isn't.
To generalize more effectively doesn't necessarily mean that radiologists need better algorithms, he continued. Instead, machine-learning developers need to better understand the data coming from MRI, and ultrasound machines, as well as CT and PET scanners. In particular, they need to understand the distribution shift of data between individual machines, and also between manufacturers. Furthermore, they should ask what parts of data distribution shift are important, understand how subtle changes in pixel data affect the features important to algorithms, and whether there are things they can do to harmonize pixel data to enable algorithms to generalize better.
The new ACR Data Science Institute is dedicated to developing tools and procedures to harmonize the clinical parts of radiology data, and to developing ways to verify that these radiology, machine-learning apps work as advertised, he added. He also provided radiologists with advice on how to manage the coming tidal wave of the many interactive machine-learning applications, each sending and receiving data from other applications.
"Complex ecosystems of cascades of multiple parallel machine-learning apps are known as dynamical networked systems. As these sorts of systems come online in radiology, this radiology machine learning software supply chain will require a new workforce with new skills. The Society for Imaging Informatics in Medicine (SIIM), along with EuSoMII and radiology societies such as the ESR, ACR, American Association of Physicists in Medicine (AAPM), and RSNA, are starting to explore what new skills are needed," Geis noted.
The Dutch perspective
Delegates also heard how imaging informatics developments are changing radiology. The specialty should play a part in this trend by training radiologists and residents in the subject, according to computer scientist Peter van Ooijen PhD, associate professor in medical imaging informatics at the Center for Medical Imaging, at the University of Groningen in the Netherlands.
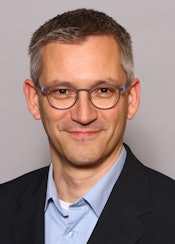
In a bid to move toward a means for subspecialization in imaging informatics for radiologists in Europe, EuSoMII has contributed dedicated sections to ESR's curriculum for radiological education, noted van Ooijen in his presentation, Preparing your team for AI. These sections are now published for undergraduate radiological education and level I and II training for radiologists.
"For the first time, imaging informatics is also included in the level II subspecialty training curriculum. This shows the importance and relevance of medical imaging informatics. Future radiology will be depending heavily on this field and therefore radiologists should start to get trained now," van Ooijen noted.
He added that radiology teams should be strengthened by including imaging informatics experts from the fields of computer science and biomedical engineering. Furthermore, radiologists should be knowledgeable about the new developments and possibilities in AI and deep learning to allow them not to be afraid of the technology but to embrace it and integrate it into their work.
"There are already resources available for learning about these new technologies and several European countries are already working on training residents and radiologists in medical imaging informatics topics," he said.
In order to play a leading role in adopting imaging informatics developments and allowing them to impact practice, radiologists must gain initial insight: Training and subspecialization, therefore, are of vital importance, he noted, pointing to how in the coming year, EuSoMII planned to collect and create educational materials, paving the way for imaging informatics to be included in the European Diploma in Radiology (EDiR) examination. The society will also be working on subcertification in imaging informatics for radiologists, he noted.