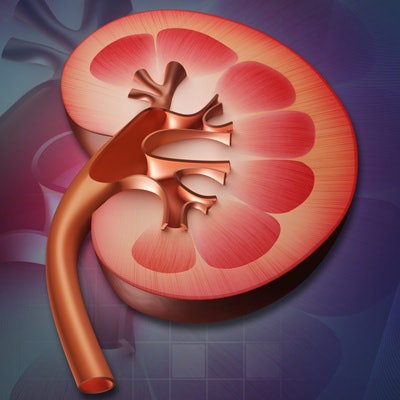
VIENNA - The combination of CT radiomics and a machine-learning algorithm can help predict if a renal cyst is benign or malignant, potentially helping to avoid unnecessary surgeries, research presented at ECR 2022 indicates.
Researchers from Maastricht University in the Netherlands developed a machine-learning algorithm to aid in classifying renal cysts based on a CT radiomics signature. In testing, the model yielded a promising level of performance.
"The proposed radiomics signature consisting of five features may prove useful for malignancy classification of renal cysts," said presenter Zohaib Salahuddin, a doctoral candidate at Maastricht University.
Renal cysts have become a frequent finding on cross-sectional imaging, especially in patients over the age of 50. However, the Bosniak classification scale -- the standard radiological criteria for malignancy risk estimation in renal cysts -- suffers from interreader variability and leads to unnecessary surgeries that can result in decreased renal function and morbidity, Salahuddin said.
"Therefore, we need to develop an interpretable quantitative image analysis technique that is accurate and reproducible for malignancy prediction of renal cysts," he noted.
In their retrospective study, the researchers first used a dataset of 264 renal cysts (247 benign and 17 malignant cysts) from the University of California, San Francisco to train and validate a machine-learning algorithm. They then used an external testing dataset of 39 lesions (24 benign and 15 malignant cysts) from Maastricht University Medical Center. Two radiologists annotated the region of interest for each cyst using software from MIM Software.
After 93 radiomics features were initially extracted from the image using the open-source Pyradiomics software, the researchers settled on five texture and first-order features to make up the radiomics "signature." They then trained their machine-learning model, which was an ensemble of linear support vector machines.
In testing on the external dataset, the researchers achieved strong performance.
Performance of machine-learning algorithm for characterizing renal cysts | ||
Benign cysts | Malignant cysts | |
Precision | 0.88 | 0.79 |
Recall | 0.84 | 0.73 |
"This is quite encouraging because a recent study showed that the performance of the radiologist using the Bosniak classification scale is only 0.76 and the interobserver agreement is only 0.77," he said.
To further investigate how the machine-learning model arrived at its results, the researchers incorporated Shapley Additive Explanations (SHAP). Summary SHAP plots are useful for revealing inconsistencies present in the model, as well as enabling the clinicians and radiologists to understand how the model is behaving, Salahuddin said.
"Post-hoc interpretability analysis may enable clinicians to utilize this radiomics signature together with the Bosniak classification scale for better sensitivity and specificity," Salahuddin said.
He acknowledged that the research did not consider the reproducibility of radiomics features due to scanner variations and segmentation variabilities. Furthermore, additional studies need to be performed, including test-retest studies to assess reproducibility as well as prospective validation to determine if the proposed radiomics signature would be effective in the clinic, he said.