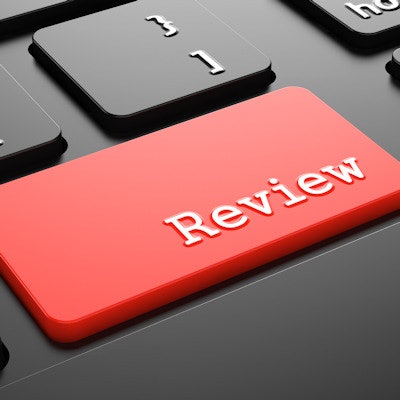
A regional radiology quality assurance (QA) system that features peer review by remote experts has improved the process of monitoring the performance of radiologists at government clinics in Moscow. It has also yielded a significant decrease in the percentage of imaging cases with discrepancies.
Many investigators have explored the topic of radiologic discrepancies, and we have to admit here has not been a significant change in the prevalence of radiologic errors since the beginning of the 20th century. Furthermore, advances in imaging technology have made the radiologist's work more complicated and increase the probability of errors.
It's hard not to agree with the opinion of A. Brady that "radiologist reporting performance cannot be perfect, and some errors are inevitable," and that "strategies exist to minimize error causes and to learn from errors made."1 The position of D.B. Larson and colleagues that the classical approach to scoring-based peer-review tends to drive radiologists against each other and practice leaders is also reasonable. They have successfully implemented a different model based on peer feedback, learning, and improvement in many radiology practices in the U.S.2
However, the assessment of radiologist performance is an important tool in the management of a large radiology department, especially if it encompasses dozens of state clinics. In addition, it is not so easy to use interpersonal professional relationships to achieve high levels of individual and organizational performance in small outpatient clinics, where usually only one radiologist works per shift. It's rare when they meet together.
In this article, we would like to share how we have addressed this challenge in Moscow with an advanced remote QA system.
Remote regional QA system
Currently, Moscow's radiology departments of 75 outpatient clinics are connected to the Unified Radiological Information Service (uRIS), a regional RIS. The radiology service comprises 130 CT and MRI units, 422 radiologists, and 321 technicians. Information from all departments is concentrated in a single center -- the Radiology Research and Practice Center of Moscow.
The integration of radiology departments into a single network made it possible to organize an electronic QA system in radiology, which includes the following:
- A tracking system for discrepancies
- Consultation on challenging diagnostic cases by a subspecialized radiologist
- A system to support learning
- Remote equipment monitoring (collecting metrics from each apparatus)
- Timely technical support
Advanced peer review
We have incorporated a number of traditional peer-review capabilities into our advanced QA system, including the following:
- An electronic discrepancy detection module (DDM). This software module is designed to choose, anonymize, and review studies, as well as provide feedback and select interesting cases for the learning system (figure 1).
- Gathering "big data" for systematic measurement and analysis of discrepancies. This analysis is made possible by the ability to connect to a large number of clinics and any PACS. An example of such an analysis is presented below (figure 2). A performance evaluation of 207 radiologists, 168 technicians, and 9,396 studies showed that the distribution of the radiology department staff's efficiency had the form of the exponential curve -- meaning that 89% of radiologists and 83% of technicians had a performance of more than 90%. As a result, activities to improve the quality of work can be concentrated only on these 11% to 17% of specialists who had lower performance.
- Outsourcing of peer review. Peer review is extra work for a radiologist, and it's known that radiologists do not like this work for many reasons. Often, quality control responsibilities lie with the head of the department, but as a rule, the department head does not have the time and tools for systematic evaluation. The results of an external independent evaluation help the head of the department to manage the staff more effectively.
- Hiring expert radiologist's to perform peer review after an objective skill assessment based on subspecialized testing. The work of experts has to be a gainful activity. When organizations use peers as the vehicle to identify incompetent radiologists, relationships between professionals are compromised because peers are placed in an adversarial position relative to each other, rending judgments that are used to determine professional competency. To preserve these relationships, professionals often perform this review unfairly.2 However, radiologists who are trained and paid for seeing discrepancies are motivated to do this work qualitatively. In addition, this avoids the influence of the review on professional relations, because the experts and the initial reporting radiologist work in different places.
- Assessment of clinically significant discrepancies by several experts. If one expert believes the discrepancy is significant, the system sends the study to another expert. If the second expert does not agree, then the study is directed for the final evaluation to the third expert of the same subspecialty. This approach enables a more objective evaluation.
- Tracking a random sample of 10% of studies as well as 30% to 40% of studies with a greater likelihood of discrepancies. These studies with a higher risk for discrepancies include, for example, CT and MRI of the larynx, and MRI of the wrist and temporomandibular joint. Radiologists with a large number of discrepancies are checked more often, while radiologists who perform well and have proved themselves are checked 2% to 3% of the time. The system selects for audit only new cases from the last few days. This allows radiologists to be informed about the error within hours or days instead of weeks or months, and prevents a negative impact on the patient's care.
- Compiling of discrepancy statistics. These statistics can be individualized for each clinic, radiologist, or radiographer to facilitate improvement. The quality control focuses on two points: technical execution (artifacts, study boundaries, patient placement, scanning technique, contrast enhancement timing and phases, and pulse sequences, etc.) and diagnostic performance (cognitive and perceptual discrepancies, errors in terminology, classification, need for follow-up, etc.) No matter the employee's discrepancy rate, it is important how it varies in dynamics (figure 3).
- No punishment or disciplinary penalty. However, there is encouragement for radiologists to perform well, including incentive rewards and posting of information on the organization's website.
- Peer feedback. Daily reports are provided to radiologists and heads of department about clinically significant discrepancies. Monthly reports are sent to heads of department about their department's performance. The reports contain detailed expert comments and recommendations for avoiding such errors in the future.
- A system for learning. The educational system includes remote and classroom curricula. Webinars are the most convenient form for analysis of typical discrepancies and studying problem radiological topics. These are recorded for free access on the official website. In 2017 (at the time of writing), 89 webinars were held and the number of participants exceeded 10,200 learners from 20 regions of Russia. In addition, 67 training courses with 1,955 learners were conducted. The system also enables appropriate cases to be identified for conferences or to serve as training aids.
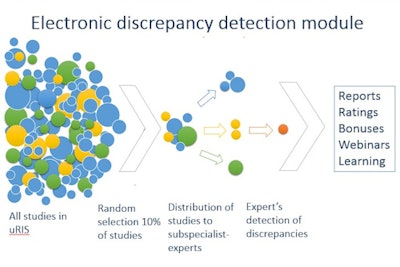
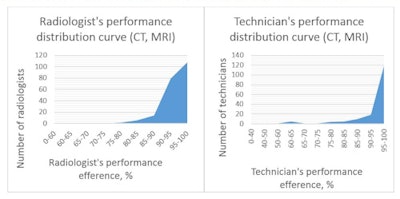
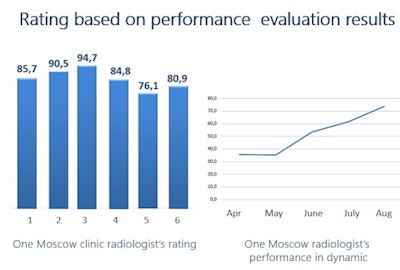
Limitations?
Unquestionably, the electronic discrepancy detection module has some limitations. For example, experts do not have the information about the case's final diagnosis, and consequently, the evaluation is just a second opinion, perhaps, of a more experienced radiologist. On the other hand, it avoids hindsight bias -- the tendency for people with knowledge of the actual outcome of an event (or presence of a radiological finding) to falsely believe they would have predicted the outcome (or seen the radiological finding).
In addition, radiologists can always appeal the results of the assessment if they disagree. This is an added motivation for radiologists to find out the final diagnosis and remember the teaching case.
The regional QA system is based on the principle of PDCA (figure 4), which stands for the following:
- Plan: set goals (to improve patient's diagnostic quality)
- Do: take actions (teaching, discrepancy webinars, equipment technical support)
- Check: quality control (advanced peer review)
- Act: analysis of causes (analytics, statistics, ranking, bid data)
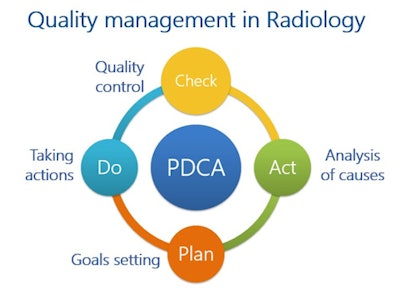
The regional remote QA system has now been in place for more than a year, and we can confidently say the system is effective for advanced peer review.
After comparing the number of discrepancies prior to the implementation of the system (1,066 CT and MRI studies in the third quarter of 2016), and after adoption (3,707 CT and MRI studies in the third quarter of 2017), we found an overall improvement in radiologist performance.
Overall radiologist performance | ||
Third quarter of 2016 (prior to adoption of remote regional QA system) | Third quarter of 2017 (after adoption of remote regional QA system) | |
Significant discrepancies | 6.4% ± 2.9% | 2.8% ± 0.8% |
Insignificant discrepancies | 19.6% ± 3% | 9.5% ± 0.7% |
Percentage of correct reports | 51.7% ± 2.9% | 69.1% ± 0.7% |
Percentage of reports with general remarks (on terminology, protocol design, etc.) | 22.4% ± 3% | 18.6% ± 1.59% |
The decrease in the number of significant and insignificant discrepancies was statistically significant (p < 0.05), as was the increase in the percentage of correct reports (p < 0.05). The difference in the number of reports with general remarks (on terminology, protocol design, etc.) was not statistically significant, however.
During this year, we conducted 2,622 consultations of complex cases, sent daily reports to heads of radiology departments regarding 484 significant discrepancies. We also sent 804 monthly department's performance reports to 67 clinics. We believe for effective quality management in radiology, it is important to maintain a balance between monitoring, support, and training -- with an emphasis on the culture of lifelong learning and development of IT systems to support radiologists.
Dr. Ekaterina Guseva, PhD, is a radiologist at the Research and Practice Center of Medical Radiology in Moscow. Dr. Natalya Ledikhova is a radiologist and head of the advisory department at the Research and Practice Center of Medical Radiology. Dr. Sergey Morozov, PhD, is CEO and professor, Research and Practice Center of Medical Radiology, and chief radiology officer of Moscow. He is also president of the European Society of Medical Imaging Informatics (EuSoMii).
References
- Brady A. Error and discrepancy in radiology: Inevitable or avoidable? Insights into Imaging. 2017;8(1):171-182.
- Larson, DB, Donnelly LF, Podberesky DJ, Merrow AC, Sharpe RE, Kruskal JB. Peer Feedback, Learning, and Improvement: Answering the Call of the Institute of Medicine Report on Diagnostic Error. Radiology. 2017;283(1):231-241.
- Reiner B. Redefining the Practice of Peer Review Through Intelligent Automation Part 1: Creation of a Standardized Methodology and Referenceable Database. Journal of Digital Imaging. 2017;30(5):530-533.
- Waite S, Scott J, Legasto A, Kolla S, Gale B, Krupinski E. Systemic error in radiology. American Journal of Roentgenology. 2017;209(3):629-639.
The comments and observations expressed herein do not necessarily reflect the opinions of AuntMinnieEurope.com, nor should they be construed as an endorsement or admonishment of any particular vendor, analyst, industry consultant, or consulting group.