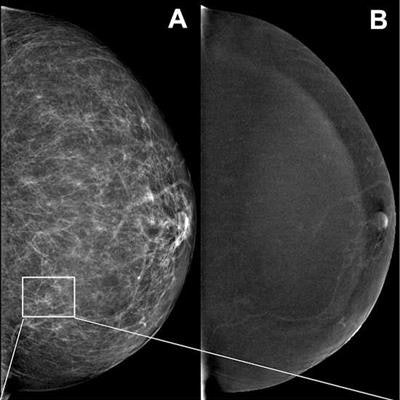
A deep-learning model can accurately identify and describe suspicious lesions on contrast-enhanced mammography (CEM) images, according to research published on 20 June in Radiology.
The study authors, led by Manon Beuque, PhD, and Dr. Marc Lobbes, PhD from Maastricht University Medical Center in the Netherlands, also found that their algorithm, when combined with handcrafted radiomics models, achieved good diagnostic performance.
"Our identification and classification model performed at a level that would make it potentially generalizable," they wrote.
While deep-learning models and handcrafted radiomics models have been shown to perform well individually for breast imaging, the researchers noted a lack of data on their combined performance.
![Example images show contrast-enhanced mammograms of a correct finding of suspicious calcifications by the deep-learning model. (A, C, D) Low-energy images in the left breast of a 58-year-old woman show a small cluster of fine calcifications (outlines [green for ground truth, yellow for prediction] in C; arrows in C and D) detected by the deep-learning model, with subtle nonmass enhancement at the site of the calcifications on the (B) recombined image. Subsequent stereotactic vacuum-assisted core-needle biopsy showed ductal carcinoma in situ (DCIS). Images and caption courtesy of RSNA.](https://img.auntminnieeurope.com/files/base/smg/all/image/2023/06/ame.2023_06_20_22_50_3281_2023_06_20_Radiology_CEM_AI.png?auto=format%2Ccompress&fit=max&q=70&w=400)
The researchers trained their deep-learning with reprocessed low-energy and recombined images for automatic lesion identification, segmentation, and classification. The researchers wanted to test their comprehensive tool on CEM images from women who are recalled. They also trained a handcrafted radiomics model to classify both human- and deep learning-segmented lesions and combined it with the deep-learning model.
The team included retrospective data from 1601 recall patients at Maastricht UMC and 283 women at Gustave Roussy Institute in Paris for the period 2013-2018. It used CEM images of suspicious breast lesions for a training set of 850 women and a test set of 212 women for the deep-learning model. The model identified 99% of lesions on an external dataset (n = 279 women).
The group found that in the external dataset, the deep-learning model achieved a lesion identification sensitivity of 90% and 99% at the image and patient level, respectively. It also achieved an average Dice coefficient of 0.71 and 0.8 at the aforementioned levels, respectively.
In addition, the team reported that the deep-learning model combined with the handcrafted radiomics model achieved an area under the curve (AUC) of 0.88 when using manual segmentations. When using deep learning-generated segmentations, the combined model showed an AUC of 0.95.
The study authors suggested that one future direction to support the model's use is to conduct a follow-up study replacing CEM images with full-field digital mammography images and compare findings.
In an accompanying editorial, Dr. Manisha Bahl and Synho Do, PhD, from the Massachusetts General Hospital wrote that with AI techniques for CEM representing "uncharted territory," further research is needed. They added that this could help address current shortcomings in this area, validate the models across different practices and CEM vendors, and assess their impact on real-world clinical practice.
The co-authors also wrote that deep-learning techniques "need not rely" on handcrafted radiomics, since incorporating such features in deep-learning models may introduce human biases.
The study can be found in its entirety here.