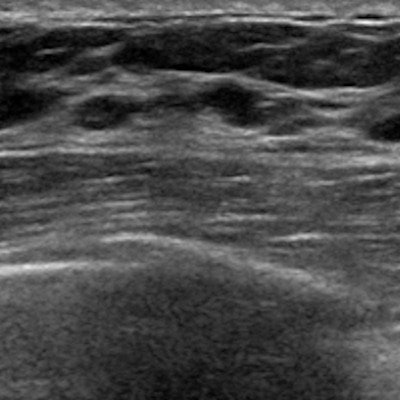
An AI algorithm may assist radiologists in improving their performance on breast ultrasound and identifying early breast cancer, according to research published on 25 May in eClinicalMedicine.
Researchers led by Jianwei Liao, PhD, from Southwest Hospital of Third Military Medical University in Chongqing, China, found that their deep-learning algorithm could help by identifying subtle elements on breast ultrasound. The model itself also achieved high diagnostic performance.
"The proposed model can extract the morphological features from early breast lesions, conduct effective and objective image analysis, and provide precise outcome for early breast cancer," Liao and colleagues wrote.
Although breast ultrasound is a go-to supplemental imaging method for confirming suspicious findings on mammography, previous reviews suggest that cancer indicators are visible on about 11% of early examinations interpreted as normal. The researchers pointed out that this may be due to factors such as noise, contrast, illumination, and resolution.
Previous studies also suggest that AI can effectively assist radiologists in breast image interpretation. In their project, Liao and colleagues developed a deep-learning model called EDL-BC to identify high-risk lesions in ultrasound images. It has two separate feature extraction modules for B-mode and Doppler images. The team wrote that it directed the model to uncover more discriminative representation from ultrasound image samples.
The researchers found that the model had high performance in both internal and external validation cohorts, marked by high area under the curve (AUC) measures. They tested the model on one internal validation cohort and two external validation cohorts. The internal cohort consisted of 7,955 lesions from 6,795 women at the First Affiliated Hospital of Army Medical University in Chongqing. The external validation cohorts included 448 lesions from 391 patients in the Tangshan People's Hospital, and 245 lesions from 235 patients in the Dazu People's Hospital, both in Chongqing.
Performance of deep learning model in training, validation sets | |||
Internal validation set | External validation set No. 1 | External validation set No. 2 | |
AUC | 0.95 | 0.956 | 0.907 |
Sensitivity | 94.4% | 100% | 80% |
The team also found that the model itself (AUC = 0.945) and the radiologists who were assisted by the model (AUC = 0.899) had higher diagnostic performance than those who had no such assistance (AUC = 0.716, p < 0.0001).
The researchers also found no significant differences between the model and radiologists with AI assistance (p = 0.099).
They made two recommendations based on the results of this study. These include highlighting that the model could improve the accuracy of diagnosis and that the model can discriminate against the high risk of early breast cancer, as well as reduce the misdiagnosis rate and avoid unnecessary invasive biopsies.
"Notably, the most crucial role of the proposed model is improving diagnostic accuracy by assisting clinicians," the study authors wrote. "The performance of this model in clinical cases shows its effectiveness in assisting the doctors to improve the diagnostic accuracy."