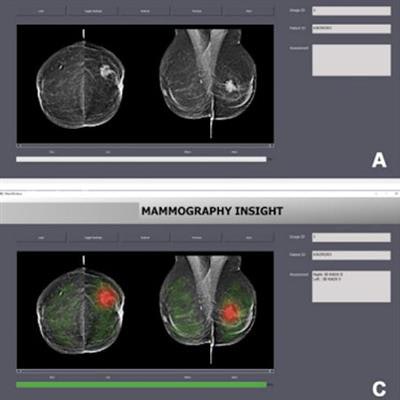
No matter the experience level, radiologists are prone to automation bias when reading mammograms while being supported by artificial intelligence (AI)-based software, suggest findings published on 2 May in Radiology.
Researchers led by Dr. Thomas Dratsch from the University of Cologne in Germany found that radiologists' performance in rating mammograms by BI-RADS category is significantly impacted by the correctness of the AI prediction.
"This information can be used by radiologists to be more critical of AI suggestions and to make sure they don't rely solely on AI support when assessing mammograms," Dratsch told AuntMinnieEurope.com.
AI and machine learning-based algorithms are seeing a surge in use by radiologists, including in breast imaging to help with diagnostic accuracy. Although previous studies suggested that radiologists and AI can work well together, there remains concern that radiologists may overrely on such decision-support systems, producing what is otherwise known as automation bias.
Two types of errors are relevant to automation bias when it comes to mammography. These include errors of commission, in which a normal mammogram is incorrectly diagnosed as having a malignancy, and errors of omission, in which a mammogram with a malignancy is incorrectly diagnosed as normal.
Dratsch and colleagues wanted to determine how automation bias can affect radiologists of various experience levels when it comes to reading mammograms with AI support.
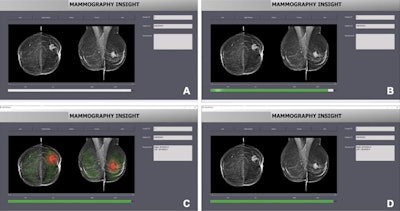
In their prospective study, the researchers had 27 radiologists of inexperienced (average, 2.8 years), moderately experienced (average, 5.4 years), and very experienced levels (average, 15.2 years) read 50 mammograms and provide their BI-RADS assessments while being assisted by an AI system. The mammograms were acquired between 2017 and 2019 and were presented in two randomized sets. These included a training set of 10 mammograms, with the correct BI-RADS category suggested by the AI system, and a set of 40 mammograms in which an incorrect BI-RADS category was suggested for 12 mammograms.
The researchers found that the radiologists were significantly impacted by the AI system's BI-RADS category predictions. The radiologists were worse at assigning the correct BI-RADS scores for cases in which the AI system suggested an incorrect BI-RADS category.
Performance of radiologists assisted by AI system in correctly rating mammograms | |||
Inexperienced radiologists | Moderately experienced radiologists | Very experienced radiologists | |
Correct predictions by AI | 79.7% | 81.3% | 82.3% |
Incorrect predictions by AI | 19.8% | 24.8% | 45.5% |
p-value | < 0.001 | < 0.001 | 0.003 |
The team also reported that inexperienced radiologists were significantly more likely to follow the AI system's suggestions when it incorrectly suggested a higher BI-RADS category compared with moderately (average degree of bias, 4 vs. 2.4) and very experienced (average degree of bias, 4 vs. 1.2) readers.
Additionally, the team reported that inexperienced readers were less confident in their own BI-RADS ratings compared with moderately and very experienced readers.
Dratsch said the team's results highlight the need for appropriate safeguards to mitigate automation bias. These include presenting users with confidence levels of the decision support system, educating users about the reasoning process, and ensuring users feel accountable for their own decisions.
He told AuntMinnie.com that future research will delve deeper into the decision-making process of radiologists using AI, possibly employing eye-tracking technology to better understand how radiologists integrate AI-provided information.
"We also aim to explore the most effective methods of presenting AI output to radiologists in a way that encourages critical engagement while avoiding the pitfalls of automation bias," he said.
In an accompanying editorial, Dr. Pascal Baltzer from the Medical University of Vienna suggested four such key strategies. These include continuous training and education, ensuring accountability for radiologists' decisions through performance benchmarking combined with continuous feedback, algorithm transparency and validation, and using AI as a triage system running in the background.
"By implementing these strategies, we can harness the potential of AI in breast imaging while minimizing the risks associated with automation bias," Baltzer wrote.