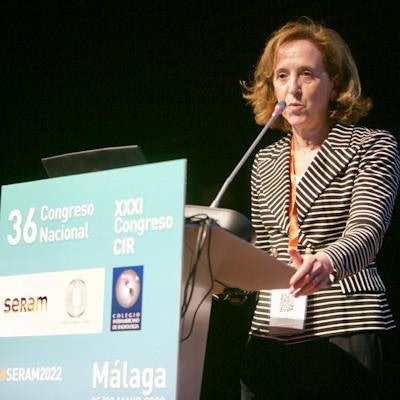
MALAGA - Lack of automation and knowledge are important obstacles to deployment of artificial intelligence (AI) in radiology practice, Prof. Dr. Laura Oleaga, PhD, said in a keynote lecture at the annual meeting of the Spanish Society of Radiology (SERAM).
Notwithstanding, great advances have been made to help radiologists diagnose disease, select treatment, monitor patients, and stratify disease risk with AI, she told delegates in a joint session organized with the RSNA.
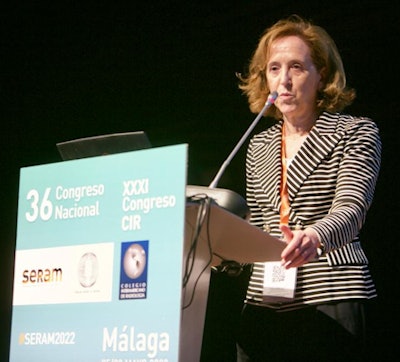
"AI enables us to extract information we can't see in an image," said Oleaga, who is former chair of radiology at the Hospital Clinic of Barcelona and scientific director of the European Diploma in Radiology (EDiR). "Data can be analyzed and combined with other data to improve diagnosis, as we can detect the development of yet invisible diseases and better assess their evaluation and prognosis."
To harness the possibilities offered by AI, radiologists must determine which solution will bring value to their departments and at what cost.
"We need to think about usefulness and purpose," she said. "We must also assess the requirements for implementation, like feasibility and maintenance costs. And, obviously, the clinical impact on patient management and treatment."
AI can also boost efficiency and productivity of radiology workflows, such as with decision-support systems that use automatized study protocols. "These strategies can help standardize protocols and improve data quality and reporting, with automatic categorization of findings and structured reporting using natural language processing and notification of incidental findings," said Oleaga, who recently completed her master's degree in AI at the Alicante AI Institute in Spain.
She has worked on organizing her own day with an AI-fed image protocolization model as part of her master's thesis. "Those tools are great to optimize workflow and save time," she said.
Clinical applications
AI solutions excel at performing high volume, low complexity tasks, such as detection of pulmonary nodules and multiple sclerosis lesions. Emphysema volume measurement tools in radiography or chest CT and algorithms that help detect pulmonary infiltrates and classify breast lesions or liver tumors are also reliable, she continued.
Oleaga and her team colleagues have trained an algorithm for detection of brain hemorrhage in acute stroke on noncontrast CT, with identification of the acute ischemic area and thrombus. The tool is far more reliable than human performance, the researchers found out.
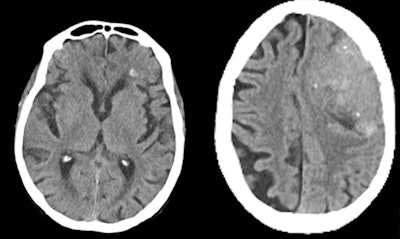
"We're not able to see the infarct at such an early stage, but the algorithm does," she said. "Such a tool can be useful in those centers who don't have the equipment to do advanced studies for stroke evaluation, to save time and make a decision to transfer the patient to centers for endovascular treatment."
AI also does well in detecting small fractures and acute incidental pulmonary thromboembolism. "Errors we make in this situation are limited, but AI is reliable, especially in oncologic patients to detect incidental thrombus at the edges of the image in abdominal studies at the pulmonary bases."
AI tools for detection and follow-up of pulmonary nodules -- with the inclusion of clinical guidelines in the algorithm, along with pulmonary emphysema volumetry and management algorithms -- are also increasingly important.
False positives
AI is certainly reliable, but it's not infallible. False positives diagnosed with AI add to the radiologist's workload, instead of taking the pain away, and this is why radiologists need to supervise all scans evaluated with algorithms, Oleaga insisted.
"Each center must validate the model they use to know when the machine fails," she said.
Another bottleneck is the lack of standardization in image processing and reporting. "We're not used to filling in structured reports and we don't label the data. That's a big limitation to AI deployment," she noted.
Automation and recording of quantitative image data are missing, and there's a lack of organization and validation of large sets. "We should work on creating large data banks that can be used for this assessment," she said.
Radiologists must improve their knowledge of AI and will require different levels of basic competence to do so. "AI needs to be included in the educational plans both at university and for residents. (...) Our role will not only be to recognize patterns but also to understand and contextualize the output of the algorithms, to know when they're working or not," she noted
To speed up AI deployment, efforts should focus on integrating the technology in workflows. "If we have to go look for the information, we're not going to use it. We're delighted with our thromboembolism and hemorrhage detection tool because it just pops in as we're doing the report. It's become a part of our daily workflow," Oleaga concluded.