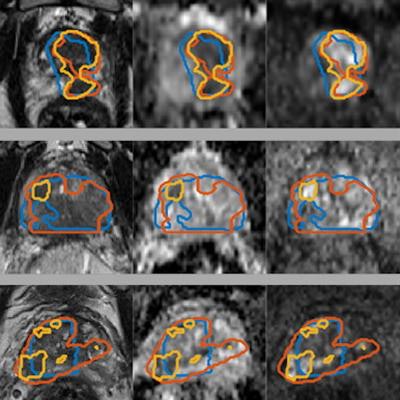
German researchers have used a convolutional neural network (CNN) to automatically segment prostate tumors in multiparametric MRI data. The CNN can produce trustworthy clinical results, even if it is trained with manually segmented data, they reported at the annual meeting of the International Society for Magnetic Resonance in Medicine (ISMRM) in London.
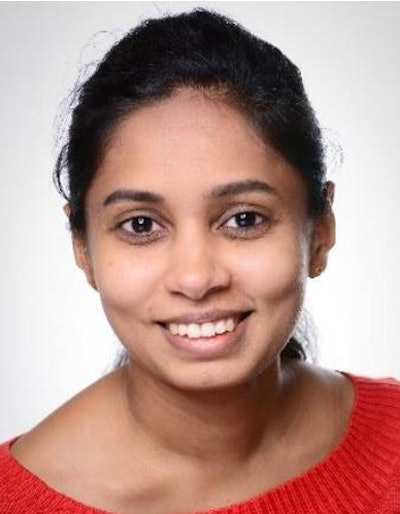
The segmentation of prostate substructures in multiparametric MRI is the basis for clinical decision-making, but due to the location and size of the gland, an accurate manual delineation of prostate carcinoma is tricky and time-consuming. Investigators at Freiburg University Hospital have shown how artificial intelligence (AI) can help in this area, and they presented their findings earlier this month in a poster at ISMRM 2022.
"So far, AI algorithms are mostly trained using contours defined by medical doctors -- in our case, radiologists and radiotherapists -- but there is always a question of how far one can trust this manually drawn ground truth to train a CNN due to the high rate of variability," lead author and doctoral student Deepa Darshini Gunashekar told AuntMinnieEurope.com.
Essentially, a CNN is a black box that generates a tumor segmentation learned from multiple MRI input data and manually drawn ground truth -- here, of the prostate, she explained.
The Freiburg team's technique
In their study, Gunashekar and colleagues in the department of radiology and medical physics at Freiburg used parametric MRI data from prostate cancer patients scanned between 2008 and 2019 on clinical 1.5-tesla and 3-tesla systems. They separated patient data into two groups: a large irradiation and prostatectomy group (n = 122), and a prostatectomy group (n = 15) from which whole organ histopathology slices were available.
For CNN training, only data from the first group were used. These exams produced precontrast T2-weighted turbo spin-echo and apparent diffusion coefficient (ADC) maps, together with computed high b-value maps (b = 1,400 s/mm²).
In the images, the entire prostate and carcinoma (gross tumor volume, GTV-Rad) were contoured by a radiation oncologist with over five years of experience, following the PI-RADs v2 standards. The researchers generated histology-based contours (GTV-Histo), and they cropped image data to a smaller field of view around the prostate gland and then registered and interpolated to an in-plane resolution of 0.78 × 0.78 x 3 mm³. They trained a patch-based 3D CNN of the U-Net architecture for the automatic segmentation of GTV and PG.
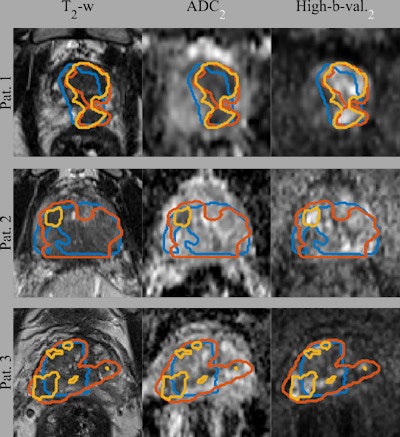
The figure shows the ground truth and the predicted segmentations overlaid on the corresponding input image sequences for three test patients from the test cohort. The mean, standard deviation, and median dice similarity coefficients (DSC) between the CNN-predicted segmentation and the ground truth across the test cohort was 0.31, 0.21, and 0.37 (range, 0-0.64) for CNN-Histo and 0.32, 0.20, and 0.33 (range, 0-0.80) for CNN-Rad, respectively.
These results indicate that the network can discriminate tumor from healthy tissue, even with training involving imperfect ground truth data, according to the authors. Increasing the database with segmentations from multiple readers might further improve the CNN as systematic biases from a single reader could be averaged out.
"The use of manually segmented ground truth data is justified for the training of CNNs for prostate carcinoma segmentation," they continued. "This is especially critical, as there cannot be any other ground truth for patients who do not undergo prostatectomy due to better, less invasive treatment options, e.g., if the tumor is curable by radiation therapy."
It is not clear, however, if the network learns to discriminate healthy from diseased tissue, or if it just learns to reproduce the experts' work, including the systematic biases. Testing the segmentation performance against radiologist-based and histology-based ground truth, the team could not find a significant difference in the segmentation.
"In this work we have a fully trustworthy ground truth data set, the whole mount histology, against which we can validate the CNN," Gunashekar said. "This is very different from what other studies usually do with AI algorithms in radiology -- here, we closely collaborated with colleagues from radiotherapy and urology to get the best possible ground truth."
The study confirms that CNNs can segment prostate tumors based on manual segmentations to the same quality as images based on histological data, she noted.
Plans for follow-up
Currently, the Freiburg team is acquiring additional data to validate the algorithm and is using explainable AI methods to further analyze which regions in the tissue contribute the most to the decisions the CNN makes. Most studies in prostate tumor segmentation achieve much lower values of the dice coefficient (a figure of merit for the quality of the segmentation) as compared with brain or kidney tumors; this could indicate that in general, it is challenging to reliably detect prostate tumors in MRI data, Gunashekar point out.
She obtained her master's degree in biomedical computing from the Technical University of Munich in 2017. She joined Freiburg as a doctoral student in 2019 under the supervision of Prof. Dr. Michael Bock, professor for experimental radiology at the University Medical Center in Freiburg. She is working on developing methods based on deep learning to address the challenges of model generalization, data variability, and quantification of the quality of CNN's prediction.