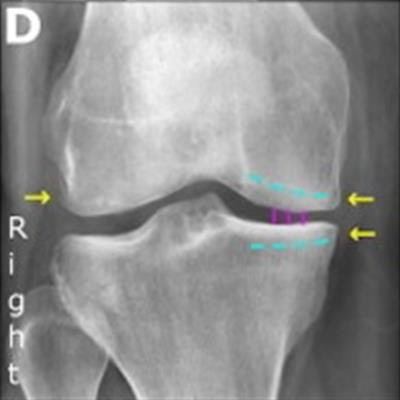
A commercially available artificial intelligence (AI) algorithm performed as well as radiologists in diagnosing the severity of knee osteoarthritis on x-rays, and it could be useful in real-world clinical settings, according to a study published on 12 March in the European Journal of Radiology.
A Danish team tested an AI program (RBknee, Radiobotics, Denmark) using a typical dataset of weight-bearing knee x-rays, as opposed to the fixed-flexion images the algorithm was trained on. The group found high agreement between the AI tool and radiologist readers, who performed almost perfectly, the authors wrote.
"Our findings support the use of the tool for decision aid in daily clinical knee osteoarthritis reporting," wrote lead investigator Dr. Mikael Boesen, PhD, head of musculoskeletal imaging and research at Bispebjerg and Frederiksberg Hospital in Denmark, and colleagues.
RBknee received the CE Mark in early 2021, with a different version of the software receiving U.S. Food and Drug Administration clearance in August 2021. The tool was designed to automatically detect findings relevant for radiographic osteoarthritis, as well as provide a secondary capture and structured text reports.
In most AI knee osteoarthritis research, x-ray images are culled from large datasets with patients in fixed-flexion positions, which provide superior information for earlier diagnosis compared with standard frontal view images of the knee joint with patients in a weight-bearing standing position, according to the authors.
Yet the diagnosis of knee osteoarthritis is mainly clinical, with weight-bearing radiography of the knee recommended as a first choice in clinical guidelines by the European Alliance of Associations for Rheumatology.
In this study, the Danish group's main goal was to evaluate the accuracy of RBknee compared with radiology professionals with varying levels of experience using a dataset of standard weight-bearing images, rather than the fixed-flexion images on which the AI tool was trained.
The researchers retrospectively enrolled 50 consecutive patients with weight-bearing, nonfixed-flexion posteroanterior radiographs of both knees (100 knees) acquired at their hospital between September 2019 and October 2019. A research radiographer reviewed all images to ensure a full range of osteoarthritis severity was covered under the Kellgren and Lawrence system, which offers five grades, from normal to severe.
Six radiology professionals were included as readers: two musculoskeletal specialists with more than 10 years of clinical experience each, two reporting technologists with more than 10 years of musculoskeletal reporting experience but no prior experience with the Kellgren and Lawrence system, and two resident radiologists. The AI tool acted as the seventh reader.
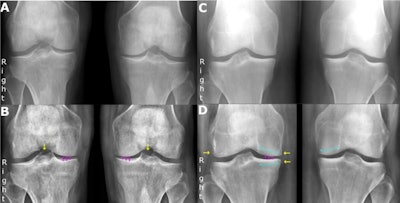
Analysis revealed agreements between the AI tool and the consensus between the two musculoskeletal specialists was 0.88, while agreement between the specialists themselves was 0.89, according to the findings.
Intrarater agreements for the specialists were 0.96 and 0.94, and 1 for the AI tool. For the AI tool, ordinal weighted accuracy was 97.8%.
The AI tool's agreement with the two reporting technologists was 0.85% and 0.82%, and agreement with the radiology residents was 0.85% and 0.85%, according to the findings.
"The AI tool achieved the same good-to-excellent agreement with the radiology consultant consensus for radiographic knee osteoarthritis severity classification as the consultants did with each other," the researchers wrote.
The researchers wrote that at their institution in Frederiksberg, knee osteoarthritis evaluation using weight-bearing radiographs of the knee is mainly performed by reporting technologists. Only rarely do the radiologists report on this type of study outside of scientific work, they wrote.
"As such, the reporting technologists are practically the daily clinical experts to some extent," they stated.
They suggested implementing the AI tool in daily clinical practice likely can reduce reading time, especially given that the program generates a report closely aligned with the type that the radiologist normally produces.
"In summary, we externally validated a commercially available artificial intelligence tool for knee osteoarthritis severity classification on a consecutive patient sample," Boesen and colleagues concluded.