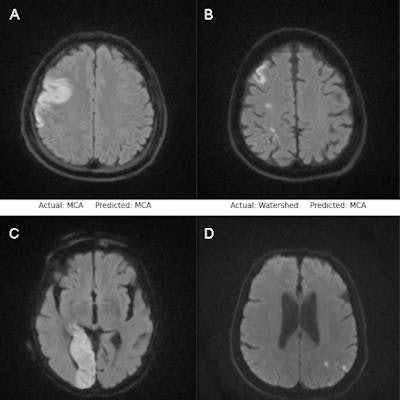
Turkish researchers have developed artificial intelligence algorithms that show considerable clinical promise for the rapid and accurate detection of stroke on MRI scans.
A group at the Batman Training and Research Hospital -- located in city the Batman in the Southeastern Anatolia Region of Turkey -- has tested algorithms based on two convolutional neural networks (CNNs) and found the models achieved high performance for both the detection of ischemic stroke and the classification of its vascular territorial type on diffusion-weighted MRI.
"Diagnosis of stroke using deep-learning methods could be particularly useful for healthcare professionals who are not familiar with stroke imaging, such as general practitioners or paramedics," wrote radiologist and first author Dr. Yusuf Kenan Cetinoglu in an article published in the December edition of the European Journal of Radiology.
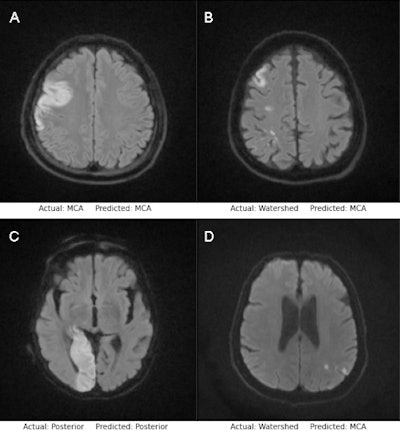
Stroke is the second most common cause of death worldwide. Delays in both diagnosis and treatment in acute ischemic stroke seriously affect brain function and increase morbidity and mortality, the researchers stated. Managing patients with ischemic stroke requires the presence of human specialists, but specialists may not always be available in every health institution.
AI techniques in stroke imaging have the potential to significantly change the diagnosis and patient management of stroke in the near future, the study authors suggested.
To that end, Cetinoglu and colleagues investigated the performance of two modified CNNs -- MobileNetV2 and EfficientNet-B0 -- for the detection of stroke as well as the areas where they occurred in the brain (vascular territories) on diffusion-weighted MRI.
The researchers culled images from 421 cases of stroke (271 acute ischemic stroke patients and 150 cases without any ischemia findings on DWI) at their hospital between January 2017 and April 2020.
They then created two custom data sets: a stroke detection data set with 1,800 slices (900 stroke and 900 normal) consisting of 1,400 for training, 200 for validation, and 200 for testing and a vascular territorial type data set with 1,717 slices (883 middle cerebral artery stroke, 416 posterior circulatory stroke, and 418 watershed stroke) consisting of 1,117 slices for training, 300 for validation, and 300 for testing.
The ground-truth label for all images was determined by consensus between two radiologist readers, one with five years of neuroradiology experience and one with 27 years of neuroradiology experience.
Both CNN models performed well, the researchers found. The MobileNetV2 model achieved 96% accuracy in detecting the presence of stroke on DWI and the EfficientNet-B0 model achieved an accuracy of 93%.
In vascular territorial classification of stroke as middle cerebral artery, posterior circulation, or watershed infarction, the MobileNetV2 model achieved an accuracy of 93% and the EfficientNet-B0 CNN model achieved an accuracy of 87%.
"Transfer learning approach with custom top CNN models achieve sufficiently high performance for both the detection of ischemic stroke and the classification of its vascular territorial type on DWI," the researchers concluded.
They noted that limits of this study included the size and diversity of the data sets. The fact that the images were obtained with a single MRI scanner was a drawback, they stated.
Nonetheless, with further studies to validate the models using large, multi-institutional data sets, diagnosis of stroke using deep-learning methods could eventually be useful for healthcare professionals who are not familiar with stroke imaging, the authors wrote.
"Our study ... might contribute to automatic stroke detection and help physicians make fast and appropriate treatment decisions," the researchers wrote.