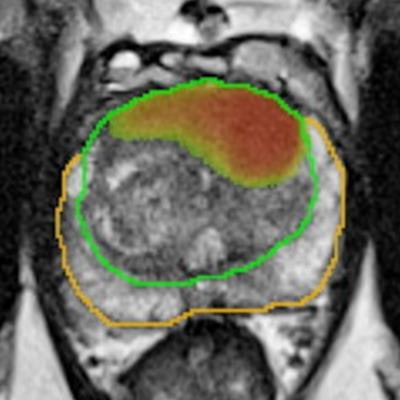
An artificial intelligence (AI)-based computer-aided detection (CAD) software application can improve the performance of radiologists in reading biparametric prostate MRI exams, according to Swiss-led research to be published in the October issue of Investigative Radiology.
In a study involving 100 prostate MRI exams and seven radiologists, a multinational research team led by Dr. David Winkel of University Hospital Basel in Switzerland found that the CAD software yielded improved diagnostic accuracy and interreader agreement when used concordantly during interpretation. What's more, it sped up median reading time by 21%.
"Such [deep learning-based] CAD software solutions may provide valuable assistance in the interpretation of prostate MRI examinations in the light of an increasing demand," the authors wrote.
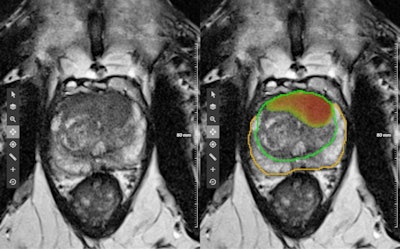
The researchers selected 100 consecutive prostate MRI cases -- with and without histopathologically confirmed prostate cancer -- from the PROSTATEx Challenge. Next, seven board-certified radiologists read each case twice over the course of two different reading sessions.
The cases and readers were divided into two groups; the first group (readers 1-4) read the first 50 cases with help from the AI software, while the second group of readers (5-7) read the same cases without assistance from the AI software. Next, the remaining 50 cases were read in an unaided manner by the first group of readers; the second group of radiologists interpreted the cases with help from the AI software.
After an interval of at least two weeks, the radiologists then read all the exams again, but this time with the other reading method. As a result, every reader interpreted every study once with and without assistance from the CAD software.
The radiologists were asked to localize and classify lesions based on version 2.0 of the Prostate Imaging Reporting and Data System (PI-RADS) and then assign a level of suspicion score on a scale of 1 (benign) to 5 (malignant) in 0.5 increments. Consensus interpretations of three experienced radiologists served as the ground truth for the study.
The study utilized a prototype of AI-CAD software (Prostate MR on syngo.via) from Siemens Healthineers. Two Siemens employees were also part of the research team.
The AI software led to better radiologist performance for detecting highly suspicious lesions.
Improved radiologist performance with use of AI software | ||
Radiologists | Radiologists with AI | |
Area under the curve for detecting clinically significant cases (PI-RADS ≥ 4) | 0.84 | 0.88 |
Interreader concordance | 0.22 | 0.36 |
Median reading time | 103 seconds | 81 seconds |
"One of the main findings of our study was that the readers benefited statistically significantly from the software solution in terms of detection and classification when a threshold of PI-RADS ≥ 4, representing highly suspicious lesions, was applied," the authors wrote.
Using a criterion of PI-RADS ≥ 3 did not produce a statistically significant increase in performance, however.
"To the best of our knowledge, this is the first rigorous study of an integrated and fully automated deep learning-based lesion detection and classification system to support the interpretation of prostate MRI in a concurrent reading scenario," the team concluded.