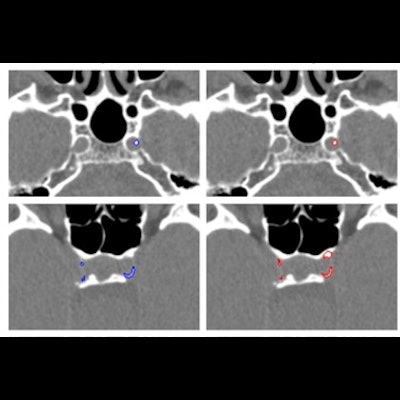
A deep-learning algorithm performs comparably to human readers when it comes to identifying intracranial carotid artery calcification (ICAC) on noncontrast CT images, according to a study published on 30 June in Radiology: Artificial Intelligence.
ICAC is an indicator of intracranial arteriosclerosis, a risk factor for stroke. The study results suggest that this kind of deep-learning model could help clinicians better track patients at risk of cerebrovascular disease by automating what can be a laborious process, wrote a team led by Gerda Bortsova of Erasmus Medical Center in Rotterdam, the Netherlands.
"Automating ICAC assessment could ... facilitate research on the etiology and clinical consequences of intracranial arteriosclerosis and may ultimately enable the use of ICAC assessment in clinical practice," the group wrote.
Intracranial carotid artery calcification is currently measured manually -- which can be time-consuming and prone to error. That's where AI could help, according to Bortsova and colleagues.
To this end, the team sought to create and validate a deep-learning-based automated ICAC algorithm for estimating stroke risk on noncontrast CT exams using data from 2,319 patients who participated in the Rotterdam study (which investigated heart failure and risk of stroke).
Patients underwent noncontrast CT exams between 2003 and 2006 and were monitored for stroke until January of 2016. For this study, two radiologist readers manually delineated the condition on the CT scans and the deep-learning model was trained to segment intracranial carotid artery calcification and quantify its volume. The model's performance was assessed by comparing the radiologist readers' manual and the model's automated segmentations with those produced by an independent observer, the group wrote.
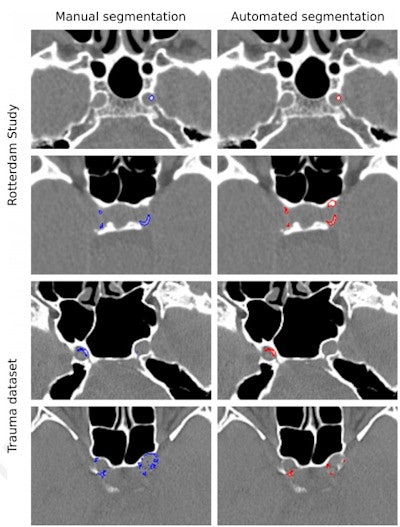
For identifying ICAC, the deep-learning algorithm showed a sensitivity of 83.8% and a positive predictive value of 88%. Correlation between manually performed and automated ICAC volume measures was 0.98. The researchers also found a strong association of ICAC volume with stroke for both automated and manually measured volumes, with hazard ratios of 1.38 and 1.48, respectively.
Radiologist and deep-learning model performance for identifying intracranial carotid artery calcification on CT exams | ||
Measure | Radiologist readers | Deep-learning model |
Sensitivity | 73.9% | 83.8% |
Positive predictive value | 89.5% | 88% |
Using deep learning for this kind of disease assessment could support radiologists coping with large datasets, according to the team.
"Automated assessment may replace manual assessment in research or clinical settings, facilitating analysis of large amounts of data," Bortsova and colleagues concluded. "Automated assessment could also be used as a starting point for manual annotations, which would speed up annotation and could increase the completeness of ICAC burden estimation."