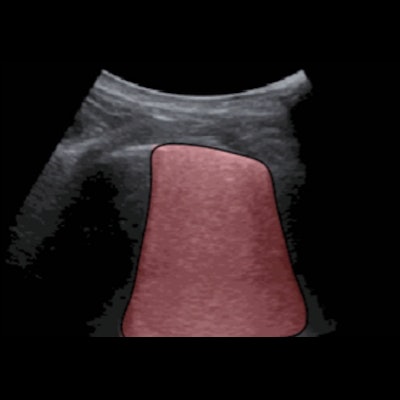
Deep-learning algorithms can be used to automatically provide risk scores on lung ultrasound exams in COVID-19 patients, researchers from Italy reported in an article published online on 27 May in the Journal of the Acoustical Society of America.
A multi-institutional team of researchers trained deep-learning models to automatically score and provide semantic segmentations of lung ultrasound exams in COVID-19 patients. In testing, the deep-learning algorithms yielded a high level of agreement with lung ultrasound experts for stratifying patients as having either low- or high risk of clinical worsening, according to the group led by corresponding author Libertario Demi, PhD, of the University of Trento.
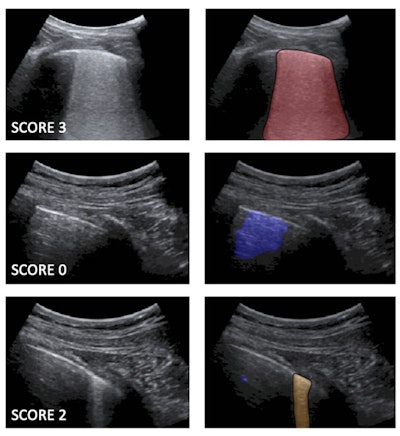
"These encouraging results demonstrate the potential of [deep-learning] models for the automatic scoring of [lung ultrasound] data, when applied to high-quality data acquired according to a standardized imaging protocol," the authors wrote.
Although lung ultrasound has been shown to be useful for evaluating COVID-19 patients, the modality is often limited to the visual interpretation of ultrasound data. As a result, there are concerns over reliability and reproducibility, according to the researchers.
Following up on prior work to develop standard lung ultrasound protocols and training deep-learning algorithms for COVID-19 patients, the researchers wanted to compare the performance of their models with that of expert clinicians. The first algorithm labels each video frame with a score, while the second provides semantic segmentation -- assigning one or more scores to each frame.
They used their algorithms to evaluate 314,879 ultrasound video frames from 1,488 lung ultrasound videos on 82 COVID-19 patients. The ultrasound exams were acquired using multiple ultrasound scanner types at the Agostino Gemelli University Hospital Foundation in Rome and the San Matteo Polyclinic Foundation in Pavia, Italy. None of the images were used in training the models.
The algorithm's scores for each frame are then used to generate an aggregate score for each video. The researchers tested two methods for producing an aggregate score: one based only on the labeled video frames and another that combined the labeled frames with the segmented frames.
These video-level scores were then compared with the risk scores provided by expert clinicians.
AI algorithm performance for risk stratifying COVID-19 patients | ||
AI risk scores based on only labeled frames | AI risk scores based on combination of labeled and segmented frames | |
Agreement with expert clinicians | 83.3% | 86% |
Although the combined approach generally outperforms the first method, the improvement isn't significant. What's more, the first method seems more stable to threshold variations, according to the researchers.
"Therefore, the first approach can be considered as a self-standing strategy to classify [lung ultrasound] videos starting from a frame-based classification," the authors wrote. "Nevertheless, the semantic segmentation remains essential for clinicians, as it provides the explainability of the decision by highlighting the specific [lung ultrasound] patterns."
In future work, the researchers plan to expand their existing database and train deep-learning algorithms on video-labeled data instead of just frame-based labeled data. That approach would be more consistent with the process used by clinicians when evaluating lung ultrasound videos, they said.