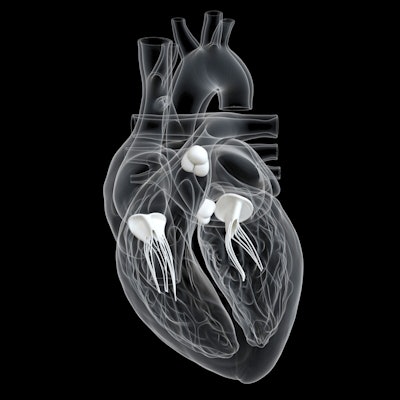
Researchers from South Korea reported that their artificial intelligence (AI) model was highly accurate for automatically detecting and quantifying aortic valve calcium on cardiac CT, even outperforming radiologist assessments in severe cases.
In a retrospective study published online on 6 February in the European Journal of Radiology, a group led by Dr. Suyon Chang of the Catholic University of Korea in Seoul outlined how its deep-learning algorithm was able to detect over 98% of cases with aortic valve calcium. What's more, the model was deemed to be superior to four radiologists for predicting severe aortic valve calcium cases.
Aortic valve calcium scores have increasingly shown clinical utility as a prognostic risk factor in patients with aortic stenosis, but motion or blooming artifacts can affect the accuracy of manual segmentation. Therefore, it can often be challenging for radiologists to distinguish aortic valve calcium from surrounding structures. Furthermore, visual assessments of aortic valve calcium grade are subjective and often inaccurate, according to the authors.
As a result, they set out to create a deep-learning algorithm to provide fully automated quantification of aortic valve calcium from noncontrast-enhanced, electrocardiogram-gated cardiac CT exams. They also wanted to compare the performance of the algorithm's quantification of aortic valve calcium and Agatston score for classifying severe cases with that of visual gradings by radiologists.
The researchers trained and validated their algorithm using 452 scans and then tested it on a separate set of 137 cases. Each CT exam was manually annotated by a radiologist with seven years of experience in cardiothoracic imaging. In comparison with these manual measurements, the deep-learning algorithm yielded 99% sensitivity but 84.2% specificity for detecting aortic valve calcium.
"Despite these false-positive cases for [aortic valve detection] detection, our [deep-learning] algorithm may be useful because it is clinically important to suggest the presence of severe [aortic valve calcium] on CT," the authors wrote.
An observer performance study was also conducted on the test set of 137 exams and included two radiology residents and two subspecialty-trained cardiothoracic radiologists. Over two reading rounds conducted two weeks apart, the readers evaluated the CT exams for the presence and grade of aortic valve calcium.
In the first round, the readers independently reviewed the CT studies for the presence or absence of aortic valve calcium and then assigned one of four grades of aortic valve calcium. In the second round, the reader utilized a visual grading system developed by the researchers for determining severity of aortic valve calcium.
Performance of AI for classifying severe aortic valve calcium | |||
Range of readers 1-4 without grading system | Range of readers 1-4 with grading system | Deep-learning algorithm | |
Sensitivity | 52.4%-87.3% | 65.1%-92.1% | 98.4% |
Specificity | 86.1%-100% | 91.7%-100% | 94.4% |
Accuracy | 69.7%-90.9% | 76.8%-91.9% | 97% |
Area under the curve | 0.762-0.923 | 0.812-0.919 | 0.964 |
The authors concluded that the deep-learning algorithm was superior to the four readers for classifying severe aortic valve calcium. They noted that accuracy may be further improved by combining the algorithm and radiologist assessment.