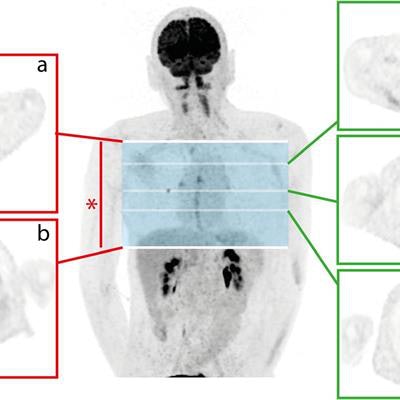
Swiss researchers have developed an artificial intelligence (AI) algorithm that could become a valuable tool for detecting small, FDG-avid pulmonary nodules on oncologic PET/CT scans, according to a study published online on 10 December in European Radiology.
More specifically, the deep-learning approach was able to boost the accuracy of nodule detection when paired with block sequential regularized expectation maximization (BSREM) reconstruction, compared with conventional ordered subset expectation maximization (OSEM) reconstruction.
"While the subjective image assessment is an important cornerstone of radiological and general imaging research, it is always hampered by the fact that it is not objective, as a matter of course," wrote lead author Dr. Moritz Schwyzer and colleagues from University Hospital Zurich. "AI may therefore potentially allow for a more reproducible and objective image analysis for various tasks (e.g., standardized image evaluation in clinical trials)."
Reliable FDG-PET
For many years, FDG-PET has been a tried-and-true way to distinguish between benign and malignant lesions based on tracer uptake and, consequently, direct patients to the most appropriate therapy. More recently, the modality has been buoyed by the introduction of a digital PET system (Discovery MI, GE Healthcare); the system features silicon-based detector technology designed to improve sensitivity and an iterative image reconstruction algorithm that incorporates BSREM to aid in the calculation of standardized uptake values (SUVs).
Concurrently, the use of AI and deep learning have come into vogue with "promising results in various medical applications, including automated detection of skin cancer, Alzheimer's disease pattern recognition on F-18 FDG PET, or lung cancer detection even at simulated ultralow-dose PET scans," the authors noted.
Considering these advances, could the combination of AI and BSREM increase FDG-PET's ability to detect small, suspicious nodules? To help answer that question, Schwyzer and colleagues looked at older oncology patients (mean age, 66 ± 11 years) who tend to have an abundance of pulmonary nodules. This patient group "calls for techniques to ensure high sensitivity and time-efficient detection of focal FDG-avid lung lesions," they added.
Unanswered questions
The researchers retrospectively analyzed 57 patients with a total of 92 FDG-avid nodules. All subjects underwent an FDG-PET/CT scan (Discovery MI) for staging or restaging between December 2017 and December 2018. The mean injected FDG dose ranged from 1.5 MBq/kg to 3.1 MBq/kg based on body weight, up to a maximum of 320 MBq. The scans showed that 37 patients (65%) had only one nodule, while the remaining 20 (35%) had two to five nodules. All nodules were 2 cm or less in size, as seen on CT.
After the PET images were acquired, the researchers performed two reconstructions: one set from OSEM with two iterations, 24 subsets, and time-of-flight and point spread function (PSF); and one set with BSREM. A total of 8,824 PET images of the lungs were extracted using both reconstruction approaches, and per-slice and per-lesion analyses were performed.
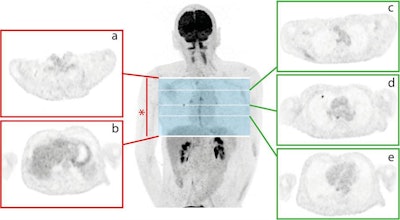
BSREM vs. OSEM
In both analyses, BSREM achieved greater sensitivity than OSEM and recorded fewer false negatives than OSEM. The 17 false-negative nodules with OSEM had a mean diameter of 8 ± 3 mm (range, 3-17 mm), while the 12 false negatives with BSREM had a mean diameter of 9 ± 4 mm (range, 3-17 mm).
In addition, the average SUVmax was significantly greater with the deep-learning algorithm and BSREM (6.1 ± 4.1), compared with the OSEM combination (4.2 ± 3.0, p < 0.001).
Diagnostic performance of deep-learning algorithm with BSREM, OSEM | |||
Sensitivity | False negatives | False positives | |
Per-slice analysis | |||
BSREM | 69.2% | 134 | 618 |
OSEM | 66.7% | 145 | 836 |
Per-lesions analysis | |||
BSREM | 87.0% | 12 | -- |
OSEM | 81.5% | 17 | -- |
To summarize, Schwyzer and colleagues listed four major findings of their study:
- Deep learning may be a valuable tool for detecting small pulmonary nodules on FDG-PET.
- SUVmax is significantly higher in small pulmonary nodules when using BSREM instead of OSEM, which translates into improved sensitivity with AI and BSREM.
- The sensitivity of deep learning for nodule detection in PET decreases when nodules have a low SUVmax or are small in size.
- The relatively high number of false-positive lesions is substantially lower in images reconstructed with BSREM than OSEM.
The authors also recommended that "future studies should target ... reducing the currently relatively high rate of false-positive findings by preprocessing PET datasets excluding extrathoracic anatomy."