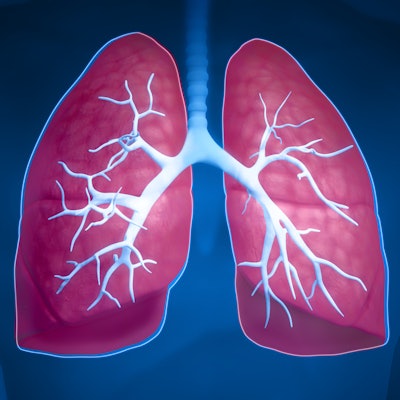
Artificial intelligence (AI)-based computer-aided detection (CAD) software can be highly sensitive for detecting pulmonary nodules on CT exams in clinical practice, according to research published on 14 August in Clinical Radiology.
Researchers from a large teaching hospital in the Netherlands retrospectively compared the performance of a commercial deep learning-based CAD software application with consensus reading of three radiologists on nearly 150 clinical cases. The algorithm yielded 88% sensitivity, which was in line with the vendor's specifications, according to the authors led by Dr. Carine Martins Jarnalo of Albert Schweitzer Ziekenhuis in Dordrecht, Netherlands.
"Together with the measured negative predictive value of 95% the system performs very well; however, these rates are still not good enough to replace the radiologist, even for the specific task of nodule detection," they wrote.
Although AI has been shown in the literature to produce high sensitivity and relatively low number of false positives in lung nodule detection, these algorithms haven't been evaluated scientifically in normal clinical workflow, according to the researchers. As a result, they sought to assess the clinical performance of a commercial deep learning-based CAD software application -- Veye Chest from Dutch AI software developer Aidence -- that has been in use at their institution for 1.5 years.
The researchers randomly selected 145 patients who had received a chest CT exam between December 2018 and May 2020 for a variety of indications. Both contrast-enhanced and unenhanced studies were included. Of the 145 exams, 97 had no nodules.
Each chest CT exam was interpreted retrospectively and independently by a radiologist with 11 years of experience and a radiology resident specializing in chest radiology with four years of experience. Both readers had access to the CAD results, as well as previously applied image annotations. Any discrepancies were resolved by a chest radiologist with 20 years of experience. These consensus results served as the reference standard for the study.
The location, composition, and maximum diameter of the nodule in the axial plane was recorded for each detected nodule by both the AI software and the radiologists.
Performance of AI software for lung nodule detection | |
Sensitivity | 88% |
Mean false positives per images per study | 1.04 |
Negative predictive value | 95% |
These results matched very well to the software's specifications of 87% sensitivity and a false-positive rate of 1.06/scan, according to the researchers.
In other findings, the researchers observed that the 11 false negatives included three calcified nodules, which have a very low risk of being malignant. The CAD software and radiologists also agreed on nodule composition in 95% of the cases.
The researchers noted some opportunities for improvement in the software, including its 40% false-positive rate. False positives in the study involved cases with atelectasis, fibrosis, and nonrounded shapes.
In addition, there "was a surprising overestimation of nodule size by the CAD system," the authors wrote. The AI software had a size discrepancy in 23 nodules; 19 (83%) were measured smaller by the radiologist.
Jarnalo and colleagues concluded that future studies should explore the software's benefit in contemporary radiological practice.
"In addition, a repeat study with a larger sample size would be of value," they wrote.