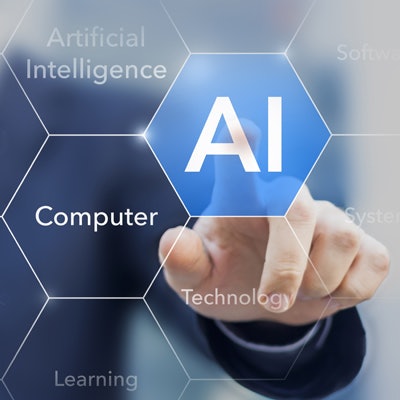
An artificial intelligence (AI) algorithm can accurately detect aortic dissection and rupture on postcontrast CT images, enabling radiologists to prioritize interpretation of these emergent cases, according to research published online on 12 September in the Journal of Digital Imaging.
A team from teleradiology services provider Virtual Radiologic led by Robert Harris, PhD, developed a convolutional neural network (CNN) to serve as an automated prescreening tool for cases containing potential aortic injuries. The algorithm was highly sensitive and specific, and helped these exams get read by radiologists minutes faster than they would otherwise.
After training, the deep-learning algorithm was evaluated on a test dataset consisting of 118 postcontrast CT series, including 50 positive studies for aortic dissection, 18 positive cases for aortic rupture, and 50 negative exams. Using a 40-mm threshold length, the model yielded 90% sensitivity, 97% specificity, and an area under the curve (AUC) of 0.979 for aortic dissections, as well as 88.9% sensitivity, 94% specificity, and an AUC of 0.990 for aortic ruptures.
Next, the algorithm was placed into clinical use. At the teleradiology group, an inference engine in the teleradiology platform first analyzes the metadata of incoming studies to determine if a study is relevant to be processed by an AI model. After assessing the study, the algorithm then sends the result back to the RIS, which adjusts the radiologist worklist to prioritize studies that likely contain the pathology.
Over a four-week period from May 17, 2019 to June 13, 2019, over 34,000 postcontrast studies were routed through the aortic injury classification model. These included 31,662 emergent studies and 3,922 trauma studies. After the researchers performed natural language processing of clinician reports to determine whether or not patients actually had an aortic dissection or rupture, they found there were seven aortic ruptures and 112 aortic dissections; the model correctly identified all seven of the aortic ruptures and 98 of the aortic dissections.
Performance of deep-learning model for classifying aortic injury | ||
Aortic dissection | Aortic rupture | |
Sensitivity | 87.8% | 96% |
Specificity | 100% | 96% |
Out of 31,662 emergent studies, 1,615 (5.1%) were prioritized by the model. In addition, the model prioritized 286 (7.3%) of 3,922 trauma studies.
"High specificity in our results was prioritized in order to keep the number of escalated studies at a minimum, as opposed to maximizing the sensitivity," they wrote. "This allows us to reduce the impact of this model on worklist, leaving room for additional pathology models to be implemented in the future."
CT studies that produced positive results on the model had a median delay -- the time gap between when their system receives a study from a facility and when it's opened by a radiologist for interpretation -- of 265 seconds, compared with 660 seconds for studies that had a negative result on the model. The 6.6 minutes of different was statistically significant (p < 0.0001).
"The model performed as expected compared with initial test data and correctly identified most dissections along with all available ruptures and reduced the time between study intake and radiologist read for these patients," the authors concluded. "This workflow can be expanded to other modalities and pathologies that are candidates for study prioritization."