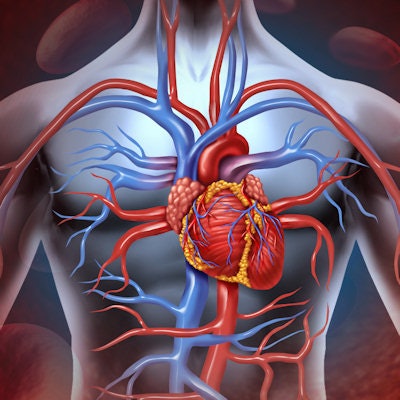
By analyzing clinical and hybrid imaging data, an artificial intelligence (AI) algorithm can predict which patients will go on to experience myocardial infarction (MI) or death during long-term follow-up, according to research from Finland presented at this week's International Conference on Nuclear Cardiology and Cardiac CT (ICNC) in Lisbon.
Researchers led by Dr. Luis Eduardo Juarez-Orozco, from the Turku PET Centre in Turku, reported that their machine-learning algorithm -- called LogitBoost -- yielded more than 95% accuracy in predicting MI or death after being trained on clinical, quantitative PET, and coronary CT angiography (CCTA) data from over 900 patients being assessed for suspected coronary artery disease (CAD).
"This should allow us to personalize treatment and ultimately lead to better outcomes for patients," said Juarez-Orozco in a statement from the European Society of Cardiology (ESC). The European Association of Cardiovascular Imaging (EACVI) of the ESC co-organized the conference with the American Society of Nuclear Cardiology and the European Association of Nuclear Medicine.
Although sequential hybrid imaging with PET/CCTA has demonstrated diagnostic and prognostic value in patients with suspected CAD, traditional prognostic analyses may be unable to fully exploit the potential of the structured clinical, PET, and CCTA data, according to the researchers. As a result, they sought to develop and implement a machine-learning workflow for analyzing clinical and hybrid PET/CT data to identify those patients who developed MI or died within six years.
They first gathered data from 951 patients with chest pain and went through the institution's standard protocol -- hybrid oxygen-15 water PET/CCTA -- for assessing CAD. After a CCTA scan provided 58 data elements related to the presence of coronary plaque, vessel narrowing, and calcification, those patients with findings suggestive of CAD also received stress myocardial perfusion PET to obtain 17 variables on blood flow. The researchers then searched the patient's electronic medical records to extract 10 clinical variables, such as patient sex, age, smoking history, and diabetes.
Over the average six-year follow-up for these patients, 24 had heart attacks, 49 died from any cause, and 109 underwent early revascularization. Using the follow-up results and the 85 clinical and imaging variables, the researchers then trained LogitBoost to predict who would go on to have an MI or die, independently from early revascularization. The researchers found that LogitBoost produced its best results when it included all 85 clinical variables in its analysis.
Performance of machine-learning algorithm for predicting MI or death | |||
Using clinical data only | Using clinical and quantitative PET data | Using clinical, quantitative PET, and CCTA data | |
Area under the curve | 0.65 | 0.69 | 0.82 |
Accuracy | 90% | 92.5% | 95.4% |
"Humans have a very hard time thinking further than three dimensions (a cube) or four dimensions (a cube through time)," Juarez-Orozco said. "The moment we jump into the fifth dimension we're lost. Our study shows that very high dimensional patterns are more useful than single dimensional patterns to predict outcomes in individuals, and for that we need machine learning."