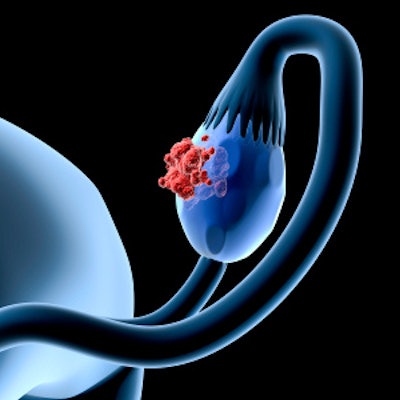
Researchers from the U.K. and Australia have developed an artificial intelligence (AI) algorithm capable of predicting the survival rate of patients with ovarian cancer by analyzing their contrast-enhanced CT scans, according to an article published online on 15 February in Nature Communications.
The group, led by senior author Eric Aboagye, PhD, from Imperial College London, tested the algorithm on hundreds of ovarian cancer cases. The team found that the tool was able to estimate the patients' likelihood of survival with as much as four times the accuracy of conventional prediction techniques.
What's more, the algorithm demonstrated a capacity to predict the patients' surgical outcomes as well as how each patient would respond to chemotherapy.
"Artificial intelligence has the potential to transform the way healthcare is delivered and improve patient outcomes," co-author Dr. Andrea Rockall said in a statement. "Our software is an example of this, and we hope that it can be used as a tool to help clinicians with how to best manage and treat patients with ovarian cancer."
AI software TexLab 2.0
The diagnosis of ovarian cancer through standard methods such as blood testing and CT scanning is often delayed until the disease progresses to its later stages, at which point the survival rate falls to less than 40%, the authors noted.
Recently, clinicians have attempted to use gene expression profiling and the examination of microRNAs to estimate an individual's risk of cancer earlier, but there are many challenges to translating such molecular biomarkers into clinically relevant information, they explained. In addition, standard methods and genetic profiling provide little insight into a patient's likelihood of survival or response to treatment.
"The long-term survival rates for patients with advanced ovarian cancer are poor despite the advancements made in cancer treatments," Aboagye said. "There is an urgent need to find new ways to treat the disease. Our technology is able to give clinicians more detailed and accurate information on how patients are likely to respond to different treatments, which could enable them to make better and more targeted treatment decisions."
Hoping to improve upon ovarian cancer prognosis, Aboagye and colleagues developed a mathematical software tool, TexLab 2.0, that uses AI algorithms to characterize tumors on CT scans. TexLab 2.0 assesses four radiomic features of tumors associated with patient survival -- tumor structure, shape, size, and genetic makeup -- and uses this information to calculate the severity of the disease. To be specific, the software extracts 657 mathematical descriptors from the scans to grade a patient's disease severity, from low risk to high risk, using a score the authors named as the radiomic prognostic vector (RPV).
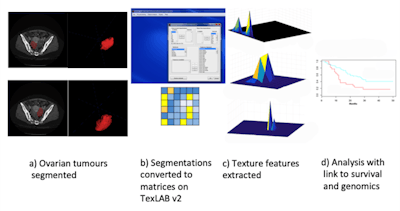
The researchers tested TexLab 2.0 in a clinical setting by using it to analyze CT scans of 364 women who presented with ovarian cancer at Hammersmith Hospital in London between 2004 and 2015.
Effective predictor
The group found that TexLab 2.0 was able to predict the survival rates of the patients with up to four times the accuracy of standard prognostic scoring techniques, including blood testing and genetic profiling. A low-risk RPV score, as calculated by TexLab 2.0, had a statistically significant association with cancer survival and proved to be an even more effective predictor of overall survival than patient age, cancer stage, and the absence of disease after surgery.
Conversely, the researchers discovered that a high-risk RPV score was associated with chemotherapy resistance and a poor surgical outcome -- highlighting the software's potential to predict how patients might respond to treatment, according to the authors. For example, patients with a high-risk score are unlikely to benefit from surgery and may need to consider an alternative approach, such as stroma modifying therapy. The software also helped them confirm that the 5% of patients who had a median likelihood of survival of less than two years had a high-risk RPV score.
The new software could help clinicians administer the best treatment to patients earlier than was previously possible and in a more personalized manner, Aboagye said. The technology may additionally enable clinicians to stratify ovarian cancer patients into different management groups based on tumor characteristics rather than on cancer stage.
In the future, Aboagye and colleagues plan to expand the scope of the study to determine the accuracy with which the software is able to predict patient outcomes after surgery, compared with drug therapy.