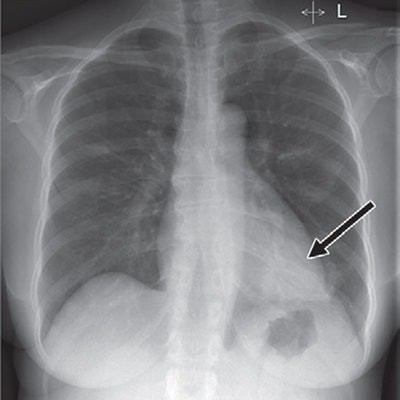
A study involving nearly 500,000 patients seen at a top London hospital over 10 years has shown that artificial intelligence (AI) can accurately identify chest x-rays that contain abnormal findings, enabling automated triage of these exams and getting them seen quicker by radiologists. The findings were published online on 22 January in Radiology.
Researchers led by Giovanni Montana, PhD, of the University of Warwick in Coventry used more than 470,000 adult chest radiographs to train an AI system that was able to identify normal chest radiographs with a 73% positive predictive value and a 99% negative predictive value. In a simulation compared with historical data, the AI system would have sharply decreased the average reporting delay for exams with critical or urgent findings.
"The results of this research show that alternative models of care, such as computer vision algorithms, could be used to greatly reduce delays in the process of identifying and acting on abnormal x-rays -- particularly for chest radiographs, which account for 40% of all diagnostic imaging performed worldwide," Montana said in a statement from the University of Warwick. "The application of these technologies also extends to many other imaging modalities including MRI and CT."
Increasing clinical demands
Radiology departments worldwide are facing increasing clinical demands that have challenged current service delivery models, and AI-led reporting of imaging could be a valuable tool for improving radiology department workflow and workforce efficiency, according to Montana.
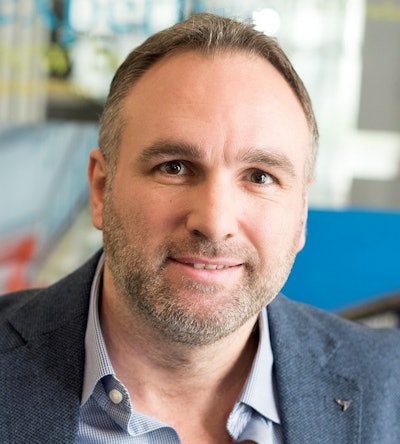
"It is no longer feasible for many radiology departments with their current staffing level to report all acquired plain radiographs in a timely manner, leading to large backlogs of unreported studies," Montana said. "In the United Kingdom, it is estimated that at any time there are over 300,000 radiographs waiting over 30 days for reporting."
The researchers hypothesized that an AI-based system could identify key findings on chest radiographs and enable real-time prioritization of abnormal studies for reporting. They set out to develop and test a system based on an ensemble of two deep convolutional neural networks (CNNs) for automated real-time triaging of adult chest radiographs based on features that could indicate they were urgent.
Montana and colleagues from King's College London first gathered 470,388 consecutive adult chest radiographs acquired from 2007 to 2017 at Guy's and St. Thomas' Hospitals in London. They divided the 413,403 studies that were performed before April 1, 2016, into training (79.7% of the studies), testing (10% of the studies), and internal validation (10.2% of the studies) datasets. The remaining exams generated after April 1, 2016, were used later in a simulation study to assess the performance of the deep-learning system in prioritizing studies.
The researchers then developed a natural language processing (NLP) system to process each of the radiology reports included in the training set and extract labels from the written text that indicated specific abnormalities were visible on the image. By inferring the structure of each written sentence, the NLP system was able to identify the presence of clinical findings, body locations, and their relationships, according to the researchers.
"The development of the NLP system for labeling chest x-rays at scale was a critical milestone in our study," Montana said in a statement from the RSNA.
Predicting clinical priority
Based on its analysis of the reports, the NLP system was able to prioritize each image as critical, urgent, nonurgent, or normal. Using these image labels, the CNNs were trained to predict the clinical priority of the x-ray images based only on their appearance. Predictions were averaged from the two different CNNs, which operated at two different spatial resolutions, to arrive at the final prediction for the system.
In testing, the system was able to separate normal from abnormal radiographs with 71% sensitivity, 95% specificity, 73% positive predictive value, and 99% negative predictive value. In terms of determining priority level, the AI system achieved 65% sensitivity, 94% specificity, 61% positive predictive value, and 99% negative predictive value for identifying critical studies.
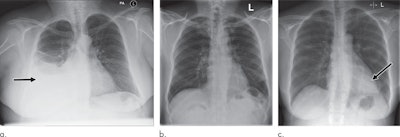
The researchers then assessed the system's performance for prioritizing radiographs in a computer simulation involving an independent set of 15,887 images. They simulated an automated radiograph prioritization system in which abnormal studies determined by the AI system to be critical or urgent could be automatically placed higher in the queue on the basis of their predicted urgency level and waiting time of other already queued radiographs.
The AI triage system would have led to a significant reduction in reporting delays of critical and urgent studies, compared with when the studies were actually interpreted, Montana and colleagues found.
Effect of AI triage algorithm on radiography reporting times | ||
Study type | Historical performance | Simulated performance with AI triage system |
Critical | 11.2 days | 2.7 days |
Urgent | 7.6 days | 4.1 days |
Nonurgent | 11.9 days | 4.4 days |
Normal | 7.5 days | 13 days |
"The initial results reported here are exciting as they demonstrate that an AI system can be successfully trained using a very large database of routinely acquired radiologic data," Montana said. "With further clinical validation, this technology is expected to reduce a radiologist's workload by a significant amount by detecting all the normal exams so more time can be spent on those requiring more attention."
Using AI to accurately triage chest x-rays and speed up the diagnosis and treatment of patients with significant illness will be "a real game-changer" worldwide, said co-author Dr. Vicky Goh, chair of clinical cancer imaging at King's College London and a consultant radiologist at Guy's and St Thomas' NHS Foundation Trust.
Future work
The researchers hope to expand their project to include a much larger sample size, as well as use more complex algorithms to achieve better performance. They would also like to prospectively assess the triaging performance of the software in a multicenter study.
"A major milestone for this research will consist in the automated generation of sentences describing the radiologic abnormalities seen in the images," Montana said. "This seems an achievable objective given the current AI technology."