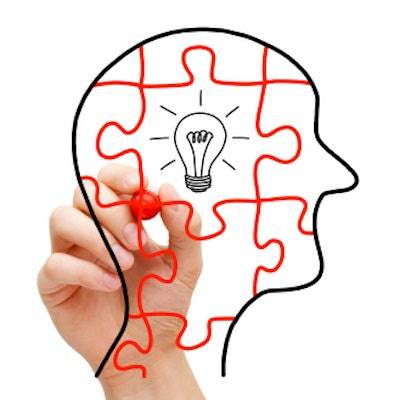
The combination of machine learning and functional MRI (fMRI) can reveal the cellular properties of brain regions, offering potential for noninvasive assessment of treatments for neurological diseases and the use of individualized therapies, according to research published online January 9 in Science Advances.
A multinational research team trained a machine-learning algorithm to automatically estimate brain cellular parameters from fMRI data and construct virtual brain models. These models revealed different cellular properties in the regions of the brain involved in sensory perception compared to those involved in internal thought and memories, concluded the group led by first author Peng Wang, PhD, and senior author Thomas Yeo, PhD, from the Singapore Institute for Neurotechnology at the National University of Singapore (NUS).
Although examining the brain at the cellular level could offer novel insights into the development and potential treatment of various neurological diseases, most human brain research to date has been limited to noninvasive means such as MRI, according to the researchers. To bridge the gap between noninvasive imaging and cellular understanding of the human brain, a number of research teams around the world have utilized biophysical brain models to simulate brain activity. However, many of these models rely on overly simplistic or false assumptions that all brain regions have the same cellular properties, according to the group.
Yeo, Wang, and colleagues from University Medical Center Utrecht in the Netherlands, Pompeu Fabra University in Barcelona, and the University of Barcelona chose instead to train a machine-learning algorithm using fMRI data from 452 participants in the Human Connectome Project. In contrast with previous work involving biophysical brain models, each brain region was allowed to have distinct cellular properties in the virtual brain models. The machine-learning algorithm was used to estimate the model parameters automatically.
"Our approach achieves a much better fit [than biophysical models] with real data," Wang said in a statement. "Furthermore, we discovered that the microscale model parameters estimated by the machine-learning algorithm reflect how the brain processes information."
They found that the brain regions involved in sensory perception exhibited cellular properties that were opposite of those involved in internal thought and memories. This spatial pattern of the human brain's cellular architecture closely reflects how the brain hierarchically processes information; this method of hierarchical processing is a key feature of both the human brain and recent advances in AI, according to the researchers.
"Our study suggests that the processing hierarchy of the brain is supported by microscale differentiation among its regions, which may provide further clues for breakthroughs in artificial intelligence," Yeo said.
The researchers said they now plan to apply their approach to examine the brain data of individual patients to better understand how individual variation in the brain's cellular architecture may relate to differences in cognitive abilities. They also hope that their results can be a step toward the development of individualized treatment plans with specific drugs or brain stimulation strategies.