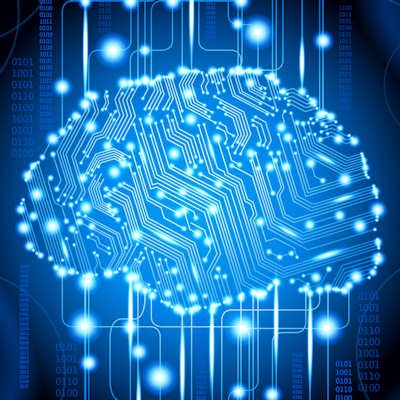
German researchers led a team that used an artificial intelligence (AI) algorithm to analyze MR images and behavioral data of people at high risk of psychosis or with depression. The algorithm was able to predict whether the individuals would need mental therapy, according to results published online on 26 September in JAMA Psychiatry.
The multinational group led by Dr. Nikolaos Koutsouleris of Ludwig Maximilian University in Munich found that the machine-learning algorithm could predict social functioning -- and thus whether therapy would be needed -- in 83% of patients in a clinically high-risk state for psychosis and in 70% of patients with depression.
"By being able to better predict what will happen to people at high risk of psychosis or with recent-onset depression over time, we are able to provide individualized treatments to clients when they first present to mental health services and potentially improve their social functioning," said co-author Stephen Wood, PhD, in a statement from Orygen, the National Centre of Excellence in Youth Mental Health, in Australia.
Previous research has found that psychosis can be predicted in high-risk individuals based on a variety of data including behavioral information and MRI scans. But whether these two disparate types of data could be combined within a single diagnostic algorithm has not been investigated, the researchers wrote.
To look into the issue further, the researchers developed the Personalized Prognostic Tools for Early Psychosis Management (PRONIA) project, in which they followed 116 people at clinical high-risk states of developing psychosis, 120 patients with recent-onset depression, and 176 healthy control participants. The patients were recruited from seven sites in five European countries.
They then used the NeuroMiner machine-learning software -- developed by Koutsouleris for the PRONIA project -- to train three types of models for predicting psychosis:
- The first model utilized behavioral information, consisting of eight baseline global functioning social and role scores.
- The second model analyzed gray-matter volume derived from MR images.
- The third model combined data from the first two into a single result.
The combination of behavioral and MRI data produced the highest level of accuracy and outperformed the predictions of expert raters, according to the researchers.
Accuracy of NeuroMiner AI for predicting social functioning outcomes | ||
Expert raters | NeuroMiner AI model | |
Patients in clinical high-risk states for psychosis | 71.8% | 82.7% |
Patients with recent-onset depression | 59.6% | 70.3% |
"These results suggest that the individualized quantification of risk for impaired functional recovery is feasible," the authors wrote.
Adding neuroimaging machine learning to clinical machine learning provided a 1.9-fold increase of prognostic certainty in uncertain cases of patients in clinical high-risk states, along with a 10.5-fold increase of prognostic certainty for patients with recent-onset depression.
"We observed pronounced benefits of blending [structural MRI] and functional data in patients with increasingly ambiguous clinical decision scores, suggesting that the cost-benefit ratio of [structural MRI] can be maximized by including it at a later, more elaborated stage of the prognostic workflow," the authors wrote.