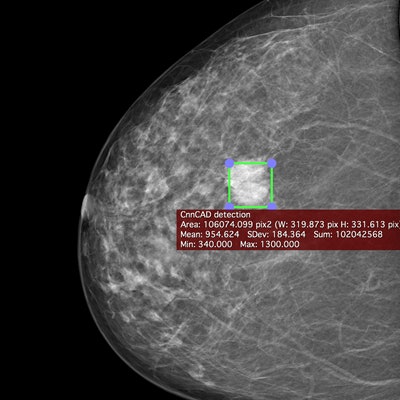
Computer-aided detection (CAD) software based on a deep-learning algorithm can accurately detect as well as characterize lesions on mammography studies, Hungarian researchers have reported in an article recently published online by Scientific Reports.
A team of researchers led by Dezső Ribli of Eötvös Loránd University in Budapest trained a deep-learning algorithm that could automatically find breast lesions and also classify them as benign and malignant. Their algorithm performed well enough to take second place in the Digital Mammography Dream Challenge, a 2016/2017 contest for developing algorithms to improve risk stratification of screening mammograms. What's more, it was also highly accurate in tests for classifying lesions in another public database of over 100 full-field digital mammography cases.
"We think that a deep-learning mammography CAD tool will be very useful for doctors," he said. "It could help improve cancer detections, and it could alleviate the pressure on radiologists that comes from the lack of specialized doctors. So we want to make it into a tool that can be used during routine diagnoses."
Deep learning revolution
Deep learning, and specifically deep convolutional neural networks (CNNs), have significantly surpassed previous computer-vision methods in the last few years, in some cases reducing error rates by tenfold, and this revolution has been fueled by high-performance graphical processing units (GPUs) and large image datasets carefully annotated by humans, according to Ribli, who is a doctoral candidate.
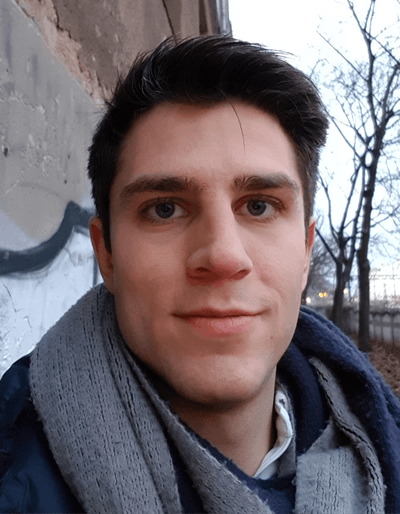
"And while these technological advances are already present in our life, (e.g., our phones analyze our pictures with deep learning), it has not been introduced to medical image analysis practice yet," he told AuntMinnieEurope.com. "Improving CAD in mammography with deep learning is especially interesting because this is one of the most challenging image analysis tasks in medicine, and current CAD solutions are under fire for their low performance and high price tags."
The researchers participated in the Digital Mammography Dream challenge, which began in 2016 and was completed in June 2017. They developed a CAD system based on Faster R-CNN, an object detection framework that's based on a VGG16 CNN with additional components for detecting, localizing, and classifying objects in an image (Sci Rep, 15 March 2018).
A different approach
Ribli noted the challenge only required algorithms to classify exams into either normal or cancer categories; algorithms did not have to identify the precise location of the cancer on the images. The database provided for algorithm training by the organizers included 640,000 mammography images that contained lesion categories, but not the precise locations of cancers. As a result, this encouraged almost all of the other teams to focus their efforts only on categorizing exams.
Knowing from experience that interpreting mammograms is very difficult, the group chose, however, to focus their effort on the localization task. Breast cancers are small, localized lesions and the exact location of the lesion is needed in order to perform further imaging, biopsy, or surgery, Ribli said.
"Therefore, a simple image classifier is almost useless because if the computer signs that there is a cancer somewhere, doctors will have to guess where [the computer found it], which may not always be possible," he said. "If [doctors] don't know what made the computer say it sees cancer, they cannot treat [the cancer]."
Because his approach focused on localizing lesions, Ribli wasn't able to use the database of images provided in the challenge for training the algorithm. Instead, he trained the model using the Digital Database for Screening Mammography, a publicly available set of 2,620 digitized screen-film mammograms that showed precisely locations of cancers. Additional training was performed with 847 full-field digital mammography images from 174 patients at Semmelweis University in Budapest.
Detection performance
The model's classification yielded an area under the curve (AUC) of 0.85 in the Digital Mammography Dream Challenge, which was good enough for second place. In addition to the AUC of 0.85 for the Digital Mammography Dream Challenge, their algorithm also produced an AUC of 0.95 for the INbreast dataset, a small dataset that includes screening and diagnostic mammograms from a European population and mammography practice. The researchers said this was highest AUC score ever produced on this dataset by a fully automated system with a single model.
On a per-lesion basis, the model produced 90% sensitivity and a false-positive rate of 0.3 false-positive marks per image, according to the researchers.
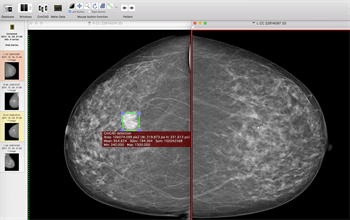
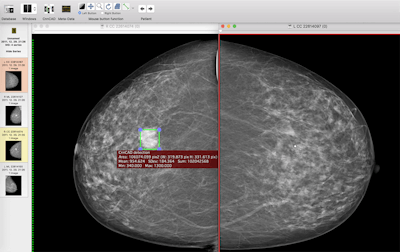
Craniocaudal view of a mammogram in left and right breast. The deep-learning-based CAD system identifies the area of the cancer (green box). All images courtesy of the Breast Research Group, INESC Porto in Portugal.
"Our model achieves slightly better detection performance on the INbreast dataset than the reported characteristics of commercial CAD systems, although it is important to note that results obtained on different datasets are not directly comparable," the authors wrote.
Ribli said the research shows the technology invented to tackle object detection in standard pictures of cats, dogs, etc., can be easily adapted to cancer detection in mammography. In addition, it already surpasses the state-of-the art for cancer detection.
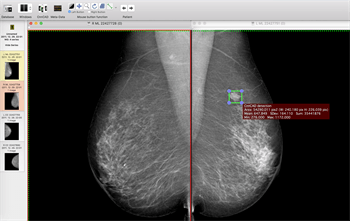
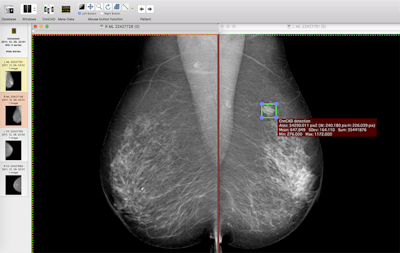
Mediolateral oblique view of a mammogram in left and right breast of the same woman. The deep-learning-based CAD system identifies the area of the cancer (green box).
"On everyday images these models are already able to rival our abilities, which were evolved for millions of years," he explained. "Our brain was not made to interpret mammograms, and perhaps deep learning can simply surpass human performance on this rather unnatural task."
Future plans
The researchers have released a demo version of their deep-learning algorithm that radiologists can try out as a plug-in for the Macintosh-bsaed OsiriX free medical image viewer. He also said that model's performance has been further improved since the Digital Mammography Dream Challenge. New results will be shared in an upcoming paper.
"Deep learning is not just a promise for medical image analysis, but it is already probably the best way to do it," Ribli said.