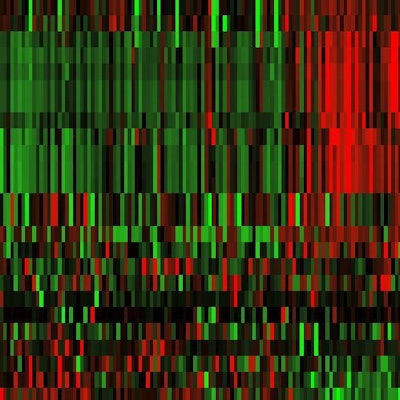
The age of big data analytics has arrived in radiology, spawning a new word: radiomics. Computer analysis of images can provide important extra diagnostic information, and more detailed descriptions of disease structures, but big data is not an end in itself and must always be measured against established radiological models, delegates will learn at the 98th German Radiology Congress (Deutscher Röntgenkongress, RöKo 2017), to be held in Leipzig from 24 to 27 May.
Many areas of clinical medicine are making increased use of big data, which consists of computer analysis of large quantities of information. Molecular medicine, genomics, and proteomics all use data from tissue and blood samples. Sophisticated analysis can make this medically meaningful, and provide useful clinical predictions.
No stone unturned
The radiological equivalent is radiomics, or computer analysis of image data.
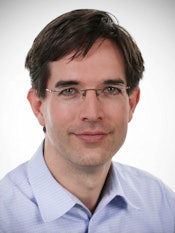
"For a long time now we've been using regional parameters, such as median values from specific parts of an image that we're particularly interested in," said Dr. David Bonekamp, a radiologist from the German Cancer Research Centre in Heidelberg, in advance of the congress. "Radiomics takes image analysis to the limits, by evaluating as many quantitative parameters as possible."
Algorithms from bioinformatics are used to extract information about the nature of the tissue being examined, its imaging phenotype, by analogy with the genotype in genomics. A comparison of genomic and radiomic parameters is a noninvasive way of making statements about the tissue's genetic properties. The data can also be used to assess the patient's prognosis or likely response to treatment: This field of radiomics is known as radiogenomics or imaging genomics.
Genomics, proteomics, and radiomics can all be used to make more precise diagnoses, and more reliable statements about likely disease outcomes and treatment responses. They aim to create more personalized medicine that takes greater account of individual patient factors in choosing the optimum therapy.
Both figures 1 and 2, for example, show a radiomic evaluation of prostate MRI data to predict whether a carcinoma will require treatment. Radiomic features have also been extracted following decimated and undecimated wavelet transformations of the originals. These include first-order characteristics such as mean values, median as well as skewness and kurtosis, and also co-occurrence and run-length-based texture features.
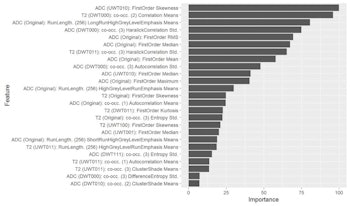
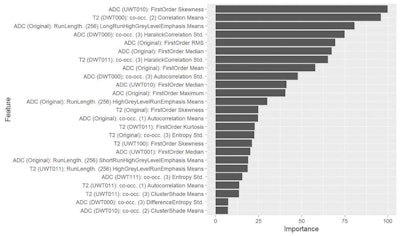
Figure 1. T2-weighted and apparent diffusion coefficient (ADC) images were analyzed. Radiomic features shown after z transformation in the form of a heat map. The red areas show a positive deviation from the mean, and the green a negative one. Patients are shown in columns and different features in times, with features being sorted by similarity. Different groups can be distinguished within the feature distribution, and then analyzed for clinically significant prostate carcinoma prediction.
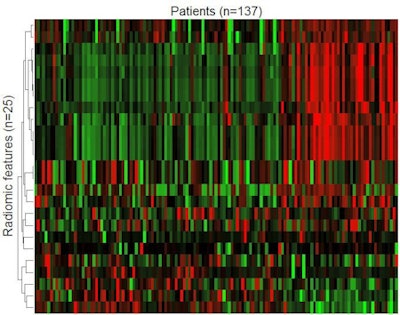
Precise tumor descriptions
In his presentation on Friday afternoon, Bonekamp plans to describe the potential and limitations of these techniques. He will use examples from cancer diagnosis: e.g., radiomic analysis has been used to develop a "signature" for glioblastomas, highly malignant brain tumors. This gives a better picture than other clinical parameters of how long the patient is likely to survive, he noted.
Another recent clinical study has evaluated the use of radiomics in breast cancer diagnosis, and specifically whether it allows better noninvasive predictions of whether tumors are benign or malignant.
"In this study, radiomics was no better than experienced radiologists," Bonekamp said. "But it was a small study with a relatively large dispersion. Future research could give different results, because big data works better the more data you include in the analysis."
Measurements against current standards
In the long-term, Bonekamp believes big data analytics could revolutionize medicine, particularly when images are analyzed by artificial neuronal networks. These are currently the most promising form of machine learning; rather than following preset analysis models, they develop their own from thousands of datasets, working in a similar way to the human brain. They have already beaten the world chess and GO (a Chinese strategy game) champions, and are being used in medicine to personalize cancer treatment. They may also transform radiology.
"It's not going to happen overnight," Bonekamp emphasized. "Radiomics is still in its infancy, and it will take time to develop."
The technology will be used clinically only if it is better than the models doctors are currently using, and he cites prostate cancer as an example.
"We already have very good radiological models, so big data has first to prove that it is better. It's not enough to be better than chance: The new methods have to be measured against current standards," he said.
Editor's note: This is an edited version of a translation of an article published in German online by the German Radiological Society (DRG, Deutsche Röntgengesellschaft). Translation by Syntacta Translation & Interpreting. To read the original article, click here.