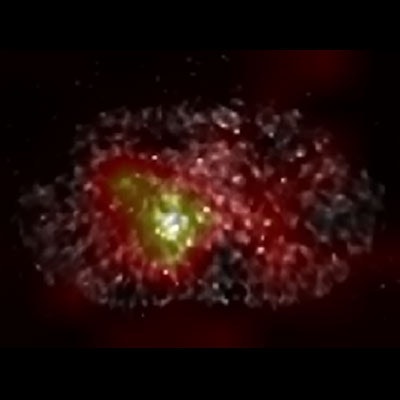
Swiss researchers have shown that machine-learning algorithms can assist fully automated FDG-PET lung cancer detection -- even at simulated very low effective radiation doses of 0.11 mSv -- and it can do so at little cost to sensitivity and specificity.
Lung cancer is one of the most frequent forms of cancer and the most common cause of cancer-related death worldwide. Therefore, techniques combined with artificial intelligence (AI) that can catch pulmonary lesions early while they are still curable will have a huge and widespread impact on patient outcomes and healthcare costs, according to the group. Further development of low-dose FDG-PET might improve the specificity of lung cancer screening and also open doors to other applications.
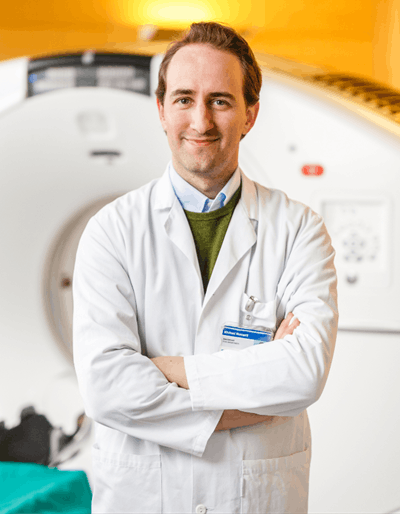
"We observed that with simulated 30-fold dose reduction in FDG dose, we had only a marginal loss in sensitivity and specificity using the AI algorithm," said supervising investigator Dr. Michael Messerli, a resident in the department of nuclear medicine at the University Hospital of Zurich. "The potential benefit of lung cancer screening is huge. These small T1 lesions 0 cm to 3 cm in size can be treated and cured, unlike the 70% to 80% of cases presenting in departments with late-stage incurable lung cancer. The long-term hope we have is that specificity of lung cancer screening can be improved by using the information from PET/CT in combination with AI. This might save costs and would have the potential to reduce follow-up appointments."
Hybrid PET/CT using F-18 FDG as a radiotracer is an established imaging method for staging of lung cancer, and now it has a role for screening, according to Messerli. In the first part of their retrospective study, the researchers assessed the accuracy of a deep-learning algorithm for automated detection of lung cancer using FDG-PET scans; in the second part of the study, they simulated a reduced FDG dose injection and evaluated its effect on the performance of the deep-learning algorithm for discriminating lung cancer (Lung Cancer, 3 November 2018).
The group assessed the FDG-PET data acquired from the PET/CT scans of 100 patients scanned for lung cancer and other malignancies during May 2017 and January 2018. Of these, 50 had histologically proven lung cancer, and 50 were clear of lung cancer or any other pulmonary lesions. The researchers studied the performance of an artificial neural network for discriminating lung cancer on a total of 3,936 PET slices that included images in which the lung tumor is visually present and image slices of patients with no lung cancer, and they performed low-dose simulation in July 2018.
The algorithm used for AI-detection was a pretrained Res-Net 34 deep neural network. The group trained it on PET data for binary classification of lung cancer/no lung cancer in July and August 2018.
Results
The group assessed the diagnostic performance of the artificial neural network based on clinical standard dose PET images (PET100%) and with a tenfold (PET10%) and 30-fold (PET3.3%) reduced radiation dose (approximately 0.11 mSv). The deep-learning network produced probabilities between lung cancer being present (1) and not present (0). The researchers plotted those probabilities on a receiver operating characteristics (ROC) curve and calculated the area under the curve (AUC). They found that the AUC of the deep-learning algorithm for lung cancer detection was 0.989 for standard dose images (PET100%), 0.983 for reduced dose PET10%, and 0.970 for PET3.3% reconstruction.
The key findings are presented in the table below:
Diagnostic performance at each Youden index for standard dose and reduced PET simulated doses | |||
PET100% | PET10% | PET3.3% | |
Sensitivity | 95.9% | 93.6% | 91.5% |
Specificity | 98.1% | 94.4% | 94.2% |
Accuracy | 97.1% | 94.1% | 93.1% |
NPV | 97.0% | 95.1% | 93.6% |
PPV | 97.5% | 92.6% | 92.3% |
Data presented as n (%). Histological findings used as reference. Data courtesy of Dr. Michael Messerli.
The artificial neural network respectively achieved sensitivities of 95.9% and 91.5% and specificities of 98.1% and 94.2% at standard dose and ultralow-dose PET 3.3%.
Speaking to AuntMinnieEurope.com, Messerli described how recent promising results from the NELSON trial mean that lung cancer screening may soon be considered as a serious proposition by many European states. The department's historical advantage of having the latest PET technology combined with the department's acquisition in 2017 of the first-ever digital PET detector, with its greater sensitivity to positron decay, contributed to the group's heightened interest in the potential of low-dose FDG-PET for lung cancer screening.
"Because the amount of tracer is detected more sensitively with this latest generation of detector, we wondered if we could lower the dose," he noted.
The researchers simulated dose reduction by unlisting the list-mode data. This enabled the group to reduce the time of PET acquisition from 150 seconds of acquired data (clinical standard) to 15 seconds (representing 10%) and then to 5 seconds (representing 3.3%) of equivalent dose-acquired data.
"In some simulated ultralow-dose images, we still could see lesions with the naked eye. However, we wanted to explore the possibility that an algorithm could learn to cope with noise better and better identify lesions," Messerli pointed out. "We also wanted to check both positive and negative cases for sensitivity and specificity: whether or not PET/CT at ultralow dose with AI caught all the real lung cancers and also whether or not it mistook normal data for lung cancer."
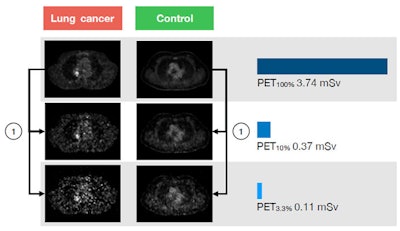
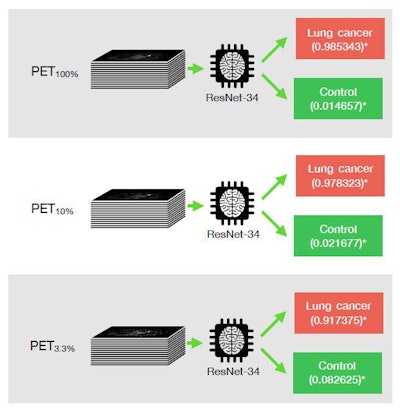
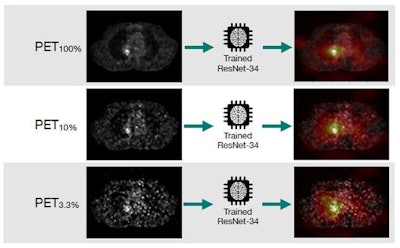
Based on the significant findings of this study, the researchers are planning a prospective study in a patient group with early lung cancer (localized stage 1a) to assess whether when rescanned with an ultralow-dose protocol and AI, they can detect early-stage lung cancer. In this study, the team also hopes to compare AI reading with experienced and less experienced human readers to see how well the algorithm performs. This planned study is still pending feasibility assessment and ethical approval.
This next study, hopefully, will also include a much larger patient dataset, ideally in a multicentric setting. Via the Achilleon Registry platform, the team has launched a European multicentric data pooling system for lung cancer FDG-PET scans. So far, the numbers on how many sites or countries will be participating are not set, but the nodule registry is already established.
Using AI algorithms to increase specificity for evaluating very-low-dose FDG-PET images to spot small malignancies and other abnormalities could also be applied to other areas such as Alzheimer's disease PET detection, notably for helping researchers determine the feasibility of a screening test to check if the brain is sick or healthy.
"We are really excited because the study shows one can use low-dose PET/CT to look at the FDG-PET data for other questions not just lung cancer. However, there is a lot of work to do before there is a clear clinical application," Messerli said.