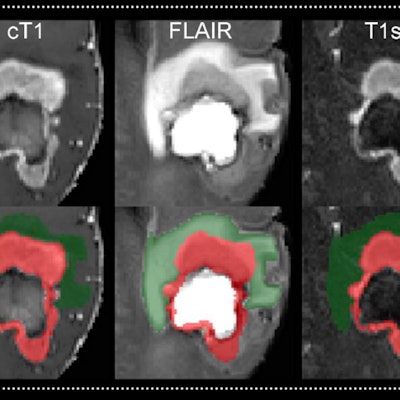
An artificial intelligence (AI) algorithm can provide automated quantitative analysis of gliomas on MRI examinations, yielding more accurate assessments of how well these brain tumors are responding to treatment than current manual methods, German researchers reported in an article published online on 2 April in Lancet Oncology.
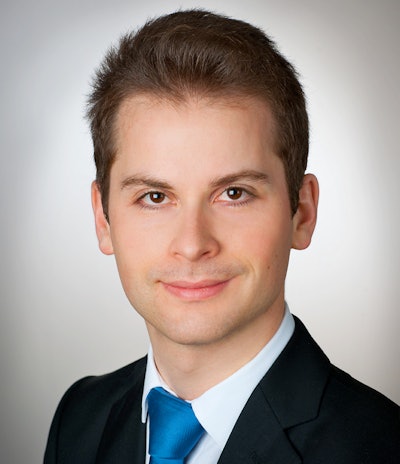
A team from Heidelberg University Hospital and the German Cancer Research Center (DKFZ) trained an artificial neural network (ANN) to recognize and localize brain tumors automatically on MRI scans, as well as volumetrically measure the tumor's individual areas and precisely assess treatment response. In testing on a large multi-institutional dataset, the ANN outperformed conventional manual measurements.
"We were able to improve the reliability of the assessment [of treatment response] by 36%," said first author Dr. Philipp Kickingereder of Heidelberg University Hospital in a statement from the DKFZ. "This can be crucial for the image-based assessment of therapy efficacy in clinical trials. The prediction of overall survival was also more precise with our new method."
Precise response assessment
Determined on MRI, the growth dynamic of brain tumors is one of the essential criteria for precisely assessing the efficacy of a new therapy. However, manual measurement of tumor expansion in two planes on contrast-enhanced MRI scans is error prone and can lead to different results.
"This can have a negative effect on the assessment of therapy response and hence the reproducibility and precision of scientific statements based on imaging," said co-author Dr. Martin Bendszus of Heidelberg University Hospital.
In an attempt to overcome the inherent limitations of manual tumor burden assessment, the researchers sought to develop an ANN-based framework to yield fully automated quantitative analysis. They first gathered a training dataset of MRI data from 455 nonconsecutive patients with brain tumors receiving treatment at Heidelberg University Hospital. They then trained an ANN to identify and localize the brain tumors, as well as volumetrically measure the contrast medium-absorbing tumor portion and peritumoral edema. It was also trained to precisely assess treatment response.
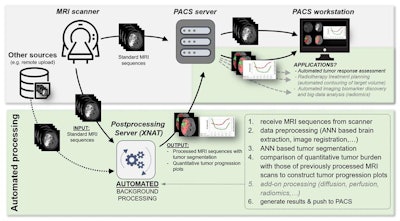
After validating and testing the ANN on internal image data, the researchers analyzed the algorithm's performance on an independent dataset from the European Organisation for Research and Treatment of Cancer (EORTC) 26101 trial, a prospective, randomized phase II/III trial of patients with first progression of a glioblastoma after standard chemoradiotherapy. This dataset included 532 patients with 2,034 individual MRI studies acquired from 16 different scanners at 34 institutions in Europe.
Excellent performance
The ANN yielded excellent performance for accurately detecting and segmenting contrast-enhancing tumors (median Dice coefficient, 0.91) and nonenhancing T2-signal abnormalities (median Dice coefficient, 0.93) on the EORTC dataset. What's more, the researchers found that quantitative assessment of tumor response via the ANN (hazard ratio, 2.59) was a significantly better (p < 0.0001) surrogate end point for predicting overall survival than manual assessment based on the Response Assessment in Neuro-Oncology (RANO) working group criteria (hazard ratio, 2.07).
To assess the variability of the ANN, the researchers determined the agreement of the automated assessment versus the radiologist ground-truth assessments for quantitative volumetrically defined time to progression. For manual assessment, the researchers compared the agreement of RANO assessments performed at local institutions with RANO assessments calculated during the EORTC trial's central review process.
The ANN had 87% agreement with radiologist ground truth, a 36% margin of improvement over the 51% agreement found between local and central RANO assessments. The difference was statistically significant (p < 0.0001).
"Overall, our results suggest that ANN can enable objective and automated assessment of tumor response and imaging biomarker discovery in neuro-oncology at high throughput, and could ultimately serve as a blueprint for the application of ANN in radiology to improve clinical decision-making," the authors concluded.
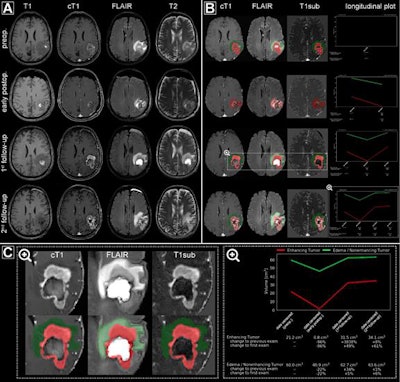
Ready for clinical use?
For now, the software is being used for research purposes only, and the researchers plan to use their technology for standardized, fully automated assessment of the treatment response of brain tumors in clinical trials. In the future, however, they would also like to see it adopted in clinical practice.
To accomplish that goal, the researchers developed and evaluated a software infrastructure that enables the integration of their method into existing radiological workflow. In testing on a simulation dataset of 466 patients with brain tumors and 595 MRI scans, the software infrastructure enabled automated on-demand processing and quantitative tumor response assessment to be completed in an average of 10 minutes per scan.
"In this way, we are creating the prerequisites for broad application and fully automated processing and analysis of MRI scans of brain tumors within a few minutes," said senior author Klaus Maier-Hein, PhD, of DKFZ in a statement.