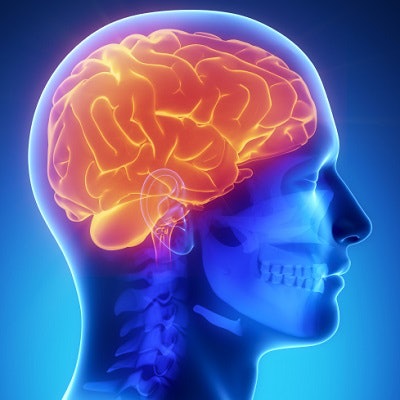
Researchers from Israel have shown the clinical potential of using automatic classification based on a single contrast of MRI to differentiate glioblastoma from brain metastasis. They've published their findings online on 11 January in the Journal of Magnetic Resonance Imaging.
Researchers led by Moran Artzi, PhD, and Idan Bressler of Tel Aviv University in Israel trained a machine-learning algorithm to perform radiomics analysis of conventional, postcontrast T1-weighted MR exams. In testing, they found that the algorithm yielded an area under the curve (AUC) of 0.96 for differentiating between glioblastoma and brain metastasis.
"The results demonstrate the clinical potential of using automatic classification based on a single contrast of MRI to differentiate [glioblastoma] from brain metastasis in newly diagnosed patients," the authors wrote.
MRI is the modality of choice for assessing patients with brain tumors, but it can be challenging for radiologists to differentiate between glioblastoma and solitary brain metastasis due to their similar appearance on MRI, according to the researchers. This task is critical, though, as glioblastoma and metastasis require different treatment strategies.
As a result, the researchers sought to apply radiomics analysis to differentiate between glioblastoma and brain metastasis subtypes based on conventional, postcontrast T1-weighted MRI. They acquired data from 439 patients: 212 with glioblastoma and 227 with brain metastasis from various origins, primarily the lung and breast. The patients had received postcontrast, 3D T1-weighted gradient-echo imaging acquired on 1.5- and 3-tesla MR systems from GE Healthcare and Siemens Healthineers. Of the patient data, 80% was used for training and 20% was set aside for testing machine-learning algorithms.
In the first phase of their radiomics analysis method, images were preprocessed and the tumor area was segmented. Next, items such as the patients' clinical information, tumor location, first- and second-order statistical features, morphological features, wavelet features, and bag-of-features were extracted, according to the researchers.
After dimension reduction was performed, the group then assessed the ability of several types of machine-learning algorithms -- support-vector machine (SVM), k-nearest neighbor, decision trees, and ensemble classifiers -- to classify the tumors.
The SVM algorithm performed the best out of the machine-learning algorithms.
Performance of SVM machine-learning algorithm | |
Differentiation between glioblastoma and brain metastasis | |
Accuracy | 85% |
Sensitivity | 86% |
Specificity | 85% |
Area under the curve (AUC) | 0.96 |
However, the performance of the algorithm varied for differentiating between glioblastoma and specific subtypes of brain metastasis, ranging from an AUC of 0.98 for glioblastoma to 0.83 for metastasis from the lung, 0.81 for metastasis from the breast, and 0.57 for other primary tumor locations.
"Classification between glioblastoma and brain metastasis subtypes may require additional MR sequences with other tissue contrasts," the group wrote.
The researchers noted that their method could easily be adapted to classify other brain tumors and could also be used to evaluate other types of machine-learning classifiers.
"This study is a step forward in developing computer-aided diagnostic tools to assist radiologists in the evaluation and classification of brain tumors," they concluded.